The 9 Best BigQuery Alternatives & Competitors in 2025
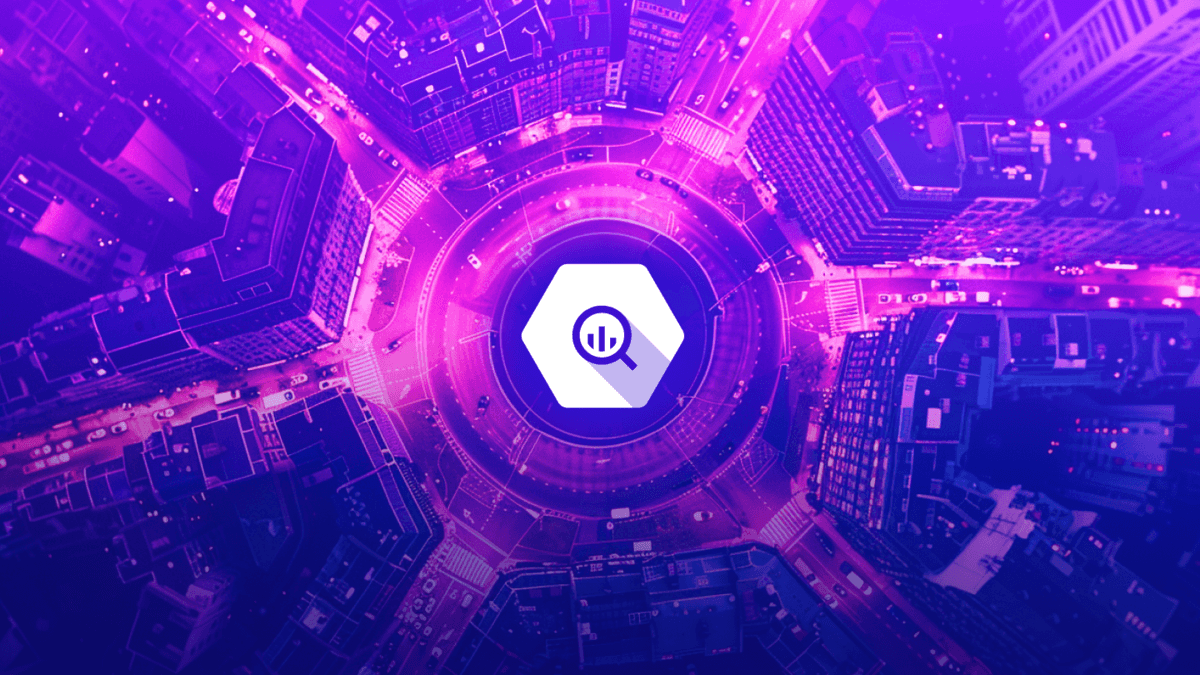
Table of Contents
BigQuery is a great warehouse—but it's not without its headaches.
If you’re a regular GBQ user, you may have run a critical query, only to get hit with unexpected delays, ballooning costs, or discovering that your data pipeline didn’t prepare your data as expected. It's a frustration many teams relying on GBQ face.
While BigQuery excels at fast SQL queries and works great within the Google Cloud ecosystem, it often falls short when it comes to managing costs and data readiness (more on this next).
In this article, let's explore the google bigquery alternatives and review the top 9 best google cloud bigquery alternatives that not only promise speed and scalability but also address these specific issues.
Top 9 BigQuery alternatives & competitors in 2025
- 5X - Best BigQuery alternative for data readiness
- Redshift - Best for AWS integration and cost-effectiveness
- Snowflake - Best for multi-cloud flexibility and automatic scaling
- Azure Synapse Analytics - Best for unified analytics and deep Azure integration
- Databricks. - Best for big data processing and collaborative data science
- IBM Db2 Warehouse - Best for advanced analytics and hybrid cloud support
- Teradata - Best for enterprise-level data warehousing
- ClickHouse - Best for real-time analytics and cost-effectiveness
- Apache Hive - Best for batch processing and Hadoop integration
Managed services
1. 5X
Call us biased but 5X is the best bigquery open source alternativeyou can find. Imagine using the power of BigQuery with other top warehouses like Snowflake and Redshift with enterprise-grade ingestion, modeling, and orchestration tools. That’s what 5X offers一all the best-of-the-breed tools in a unified workspace.
One of the most important metrics we pay attention to is "Data Readiness". Data readiness implies pulling data from different sources, centralizing it, cleaning, modeling, and structuring it to make it business-ready.
Data readiness has traditionally powered BI, reporting, analytics, and data activation (popularly called Reverse ETL). However, in the new world of AI, data readiness is the key component that powers the entire Gen AI and LLM world. If you don't understand your data, neither will AI.
The five layers of data readiness system are:
- Ingestion
- Warehouse
- Modeling
- Orchestration
- Business intelligence
How do GBQ and 5X stack up on these? Let’s find out.
Benefits of choosing 5X over other warehouses
- Quick setup: End-to-end, integrated data platform that gets you up & running in hours
- Built for business ROI: 5X maximizes ROI by boosting data team productivity & reducing infra spends by 30%
- Easy to use & scale: A single interface to build & monitor data pipelines. No hassle of switching vendors.
- Maintenance-free: 5X manages the data platform, removing the hassle of maintaining the data infrastructure
Who is 5X for
- Data-driven companies looking to simplify their data operations and eliminate complexity.
- Teams struggling with fragmented tools and high costs from managing multiple vendors.
- Businesses looking to boost ROI by reducing infrastructure costs and increasing data team productivity.
2. Redshift
Amazon Redshift stands out as the leading bigquery alternative in aws, offeringfully managed data warehouse service that allows you to run complex queries on large datasets quickly. It features high-speed querying, scalable storage, and easy integration with other AWS services. Redshift's advanced compression and data distribution techniques help manage and optimize performance, making it ideal for big companies, AWS users, data teams, and budget-conscious businesses.
Why Redshift is a good GBQ alternative
- Seamless AWS integration: Redshift integrates smoothly with other AWS services, making it a strong choice for those already using the AWS ecosystem.
- Scalability: It can handle large volumes of data, scaling as your needs grow.
- Cost-effective: Offers flexible pricing, especially with reserved instances, which can significantly reduce costs.
- Strong performance: Delivers fast query execution for complex, large-scale datasets.
- Familiar SQL interface: Provides a user-friendly experience for teams accustomed to SQL-based tools.
Who is Redshift for
- Large enterprises: Businesses that need a scalable solution to handle and analyze a lot of data.
- AWS users: Companies already using Amazon's cloud services who want everything to work together smoothly.
- Budget-minded businesses: Organizations looking for flexible and cost-effective data storage options.
Pros
- Fast query execution and scalability.
- Works well with other AWS services and offers automated backups.
- Simple to use and scale, with good documentation and auto prompts.
- Columnar storage, parallel processing, and serverless options.
- Serverless and datashare functionality, as well as a strong ecosystem and community support.
Cons
- Requires cluster management and has occasional restarts.
- High costs and complex scaling for smaller startups.
- Limited UI and query editor features, poor integration with some tools.
- Lack of cost transparency and difficulty in analyzing cluster activities.
- Missing some functions, such as JSON support and detailed ER diagrams.
3. Snowflake
Snowflake is a cloud-native data warehousing platform that offers unique features such as independent scaling of compute and storage, automatic performance tuning, and support for structured and semi-structured data. Its architecture allows seamless data sharing and collaboration across organizations while integrating with major cloud providers (AWS, Azure, Google Cloud) for maximum flexibility and ease of use.
Why Snowflake is a good BigQuery alternative
- Multi-cloud flexibility: Snowflake operates across major cloud platforms (AWS, Azure, Google Cloud), providing flexibility in cloud service choices.
- Automatic scaling: It adjusts compute and storage resources automatically based on workload, ensuring optimal performance without manual intervention.
- Performance efficiency: Snowflake delivers fast query execution with features like automatic indexing and optimized data storage.
- Ease of use: Offers a user-friendly interface and SQL support that simplifies data management and analysis.
- Cost control: Features pay-as-you-go pricing and data sharing options to help manage and optimize costs effectively.
Who is Snowflake for
- Businesses of all sizes: Suitable for small to large companies looking for scalable and flexible data warehousing solutions.
- Multi-cloud users: Ideal for organizations that use or plan to use multiple cloud platforms.
- Data-driven teams: Teams looking for efficient and performant data warehousing with minimal administrative overhead.
Pros
- Separates storage and compute, allowing for independent scaling of compute resources
- Administration tasks are automated, and the platform uses SQL, reducing the learning curve for users.
- Intuitive query writing and user-friendly interface, including integration with SSO for secure logins.
- Excellent pre- and post-sales support, and evolving feature set.
- Ability to open multiple query editors in the same window.
Cons
- The UI could be improved, users feel the need for a desktop client and difficulty in viewing metadata.
- While the pay-as-you-go model is flexible, heavy workloads can result in high costs.
- The platform may not be ideal for unstructured data, such as large volumes of JSON or similar formats.
- May encounter issues with the responsiveness of the UI on smaller screens, which can hinder usability.
4. Azure Synapse Analytics
Azure Synapse Analytics is an integrated analytics service that combines big data and data warehousing capabilities. It provides a unified experience for ingesting, preparing, managing, and serving data for immediate business intelligence and machine learning needs. With its deep integration with other Azure services, Synapse offers seamless data processing and analytics at scale, making it a compelling option for enterprises invested in the Microsoft ecosystem.
Why Azure Synapse Analytics is a good BigQuery alternative
- Unified analytics platform: Synapse combines data warehousing, big data analytics, and data integration in a single service, eliminating the need for multiple tools.
- Deep Azure integration: Seamlessly integrates with Azure services like Power BI, Azure Machine Learning, and Azure Data Lake, creating a powerful ecosystem for end-to-end analytics.
- On-demand scalability: Offers the ability to scale resources dynamically based on workload, ensuring cost-effective performance without compromising speed.
- Hybrid data capability: Supports both on-premises and cloud-based data sources, making it ideal for organizations with hybrid data environments.
Who is Azure Synapse Analytics for
- Enterprises in the Microsoft ecosystem: Ideal for organizations heavily invested in Azure and Microsoft tools, looking for seamless integration across their data and analytics services.
- Large-scale data users: Suited for companies that need to handle and analyze massive datasets with high performance.
- Hybrid cloud users: Beneficial for organizations operating in both on-premises and cloud environments, offering flexibility in data management.
Pros
- Similar to SQL Server, making it familiar for those with SQL Server experience.
- Allows T-SQL on external files, simplifying data parsing.
- Easy and fast initial configuration with no infrastructure involved.
- Provides a single portal for all needs, leveraging multiple resources on a single page without requiring a desktop-installed tool.
- Power BI datasets can be integrated, providing robust analytics capabilities.
Cons
- High coding and knowledge requirements for traditional data warehouse system features.
- Not stable enough for production use, leading to service level agreement (SLA) issues.
- Requires separate knowledge of Azure Active Directory and Azure Storage.
- Users unfamiliar with the platform may inadvertently generate high costs by activating expensive features.
5. Databricks
Databricks is a unified data analytics platform that combines big data processing, machine learning, and collaborative data science. Built on top of Apache Spark, Databricks offers a highly scalable environment for processing large volumes of data with speed and efficiency. It also provides robust integration with major cloud platforms, enabling seamless data processing and advanced analytics in a collaborative workspace.
Why Databricks is a good BigQuery alternative
- Apache Spark integration: Databricks is built on Apache Spark, offering powerful big data processing capabilities that are highly optimized for performance.
- Collaborative environment: Provides a collaborative workspace with interactive notebooks that support multiple programming languages, making it ideal for data science teams.
- Scalability: Databricks offers dynamic scaling, allowing organizations to manage workloads efficiently while controlling costs.
- Machine Learning integration: Integrated with machine learning libraries and tools, making it a strong choice for teams focused on predictive analytics and AI.
- Multi-cloud support: Operates across AWS, Azure, and Google Cloud, offering flexibility in choosing cloud service providers.
Who is Databricks for
- Data science teams: Ideal for teams that require a collaborative environment with support for multiple languages and advanced analytics.
- Big data users: Suited for organizations dealing with large-scale data processing and analysis.
- AI/ML-focused Organizations: Best for businesses looking to integrate machine learning and AI into their data workflows.
- Multi-cloud enterprises: Beneficial for companies that want to leverage the flexibility of operating across multiple cloud platforms.
Pros
- Scalable clusters that meet diverse computing needs.
- Seamless integration with Apache Spark, enhancing data processing capabilities.
- Integrated data lake, simplifying data investigation and machine learning workflows.
- Robust security protocols with role-based access control, aiding in cost management.
- User-friendly interface with strong support for open-source file formats.
Cons
- High costs and challenging contract negotiations, particularly in comparison to other solutions.
- Occasional performance issues, especially with complex workloads.
- Migrating libraries between different workspaces can be problematic.
- Cluster optimization process is not transparent, partly due to the proprietary nature of the platform.
6. IBM Db2 Warehouse
IBM Db2 Warehouse is a scalable data warehousing solution designed for high-performance analytics and advanced data management. It offers a range of features to handle large volumes of data with speed and efficiency. Built to support both on-premises and cloud environments, IBM Db2 Warehouse provides powerful capabilities for data processing, integration, and analytics.
Why IBM Db2 Warehouse is a good AWS Bigquery Alternative
- Advanced analytics: IBM Db2 Warehouse supports advanced analytics and in-database processing, allowing for complex queries and data manipulation without needing to move data.
- Performance optimization: Utilizes high-performance data processing techniques to ensure fast query execution and efficient handling of large datasets.
- Scalability: Offers dynamic scaling capabilities to manage varying data workloads effectively, ensuring optimal performance under different conditions.
- Integration capabilities: Provides robust integration with various data sources, including cloud and on-premises systems, making it versatile for diverse data environments.
- Hybrid cloud support: Operates in both cloud and on-premises environments, offering flexibility for businesses with hybrid or multi-cloud strategies.
Who is IBM Db2 Warehouse for
- Enterprise data teams: Ideal for organizations needing a high-performance data warehouse capable of handling large-scale data and complex queries.
- Data analysts and scientists: Suitable for users looking for advanced analytics and in-database processing to derive insights from large datasets.
- Businesses with hybrid cloud needs: Beneficial for companies that operate in hybrid or multi-cloud environments and need seamless integration across platforms.
Pros
- High availability features like pureScale ensure continuous operation.
- Strong SQL optimizer supports high-concurrency complex queries.
- Exceptional technical support from IBM is timely and professional.
- Handles increasing data volumes and complex datasets easily.
- Integrates well with various systems and offers a wide range of APIs for developers.
Cons
- Installation process can be complex and may require reinstallation if issues occur.
- Perpetual license requires additional yearly investment for support.
- Lacks automated query and alert features.
- Product documentation can be difficult to navigate and could benefit from more localization.
- Expensive with limited support for open source bigquary applications.
7. Teradata
Teradata is a relational database management system specifically designed for enterprise-level data warehousing and large-scale analytics. It’s built to handle extremely large volumes of data and supports complex queries with high efficiency, making it a go-to solution for organizations that require robust data processing capabilities.
Why Teradata is a strong alternative to BigQuery
- Advanced analytics: Enables detailed, in-depth analysis within the platform, eliminating the need for data movement.
- Optimized performance: Designed for fast query execution, even under heavy workloads.
- Scalability: Easily scales to meet growing data demands without performance loss.
- Integration: Connects smoothly with diverse data sources and business applications.
- Flexible deployment: Supports hybrid environments, balancing cloud and on-premises infrastructure.
Who is Teradata for
- Large enterprises: Handling vast data volumes with high-performance analytics.
- Complex analytics Needs: Businesses requiring detailed, resource-intensive data processing.
- Hybrid or multi-cloud environments: Deployments across both on-premises and cloud platforms.
- Real-time data processing: Industries needing immediate data insights for operations.
Pros
- MPP architecture enables efficient handling of large data and complex queries.
- Supports vertical and horizontal scaling with minimal downtime.
- Provides robust fault tolerance and disaster recovery.
- Integrates advanced analytics functions directly within the data warehouse.
- Offers extensive data management features for integration and governance.
Cons
- Total investment is high, making it expensive for SMBs.
- Needs improvement in agility and responsiveness of technical support.
- Configuration and management can be complex, particularly for scalability.
- Lacks frequent updates on new product capabilities and their impact on existing users.
- Some lab cases can take a significant amount of time to complete.
Open-source Bigquery Competitors
8. ClickHouse
When comparing clickhouse vs google bigquery for analytics, ClickHouse emerges as an open-source columnar database management system optimized for real-time analytical queries, offering significant cost advantages.
Why ClickHouse is a good BigQuery alternative
- Real-time analytics: ClickHouse excels in real-time data processing and complex analytical queries, offering fast performance for large datasets.
- Columnar storage: Utilizes columnar storage to optimize read and query performance, especially for analytical workloads.
- Scalability: Designed to scale horizontally, enabling efficient management of growing data volumes and high query loads.
- High performance: Implements advanced compression techniques and parallel processing to deliver rapid query execution and efficient data handling.
- Open-source flexibility: Being open-source, ClickHouse provides cost-effective scalability and customization opportunities, along with a strong community for support and innovation.
Who is ClickHouse for
- Data analysts and scientists: Ideal for users needing high-speed, real-time analytics and complex querying capabilities.
- Large-scale data environments: Suitable for organizations dealing with massive datasets and requiring efficient, high-performance data processing.
- Real-time data applications: Best for businesses that need real-time data insights and quick analytical responses.
- Cost-conscious enterprises: Beneficial for companies seeking a high-performance data warehouse solution without the cost of proprietary software.
Pros
- Fast for large-scale analytical queries due to columnar storage and optimization.
- Efficient data compression reduces storage needs and boosts performance.
- Scalable both horizontally and vertically.
- Includes versatile engines like MergeTree for efficient data handling.
- Real-time data aggregation and insights.
- Cost-effective and open-source.
Cons
- Lacks SSO support with some identity providers.
- SQL Console lacks advanced features like visual query plans.
- Limited role management in the cloud version.
- Not suited for transactional or complex multi-column queries.
9. Apache Hive
Apache Hive is a data warehouse infrastructure built on top of Hadoop, designed for querying and managing large datasets stored in Hadoop's HDFS. Hive provides an SQL-like interface for querying data, making it accessible to users familiar with traditional relational databases. Its architecture supports batch processing and complex queries over vast amounts of data, integrating well with other Hadoop ecosystem components.
Why Apache Hive is a good BigQuery alternative
- SQL-like querying: Hive's use of HiveQL, an SQL-like query language, simplifies the process of writing and managing complex queries over large datasets.
- Integration with Hadoop: Leverages Hadoop's distributed computing capabilities for handling large-scale data processing, ensuring high scalability and fault tolerance.
- Scalability: Built to scale out with Hadoop clusters, making it suitable for managing massive volumes of data and high query loads.
- Batch processing: Optimized for batch processing workloads, enabling efficient handling of large datasets and complex queries.
- Extensible: Supports custom user-defined functions (UDFs) and integration with various Hadoop ecosystem tools, providing flexibility and extensibility.
Who is Apache Hive for
- Hadoop ecosystem users: Suitable for organizations already using Hadoop for distributed storage and processing.
- Organizations with existing Hadoop infrastructure: Beneficial for businesses looking to leverage their Hadoop investments with a robust querying and management tool.
Pros
- HiveQL, similar to SQL, simplifies querying and managing large datasets.
- Works seamlessly with Hadoop for distributed storage and processing.
- Designed to scale out with Hadoop clusters, handling massive datasets efficiently.
- Optimized for batch processing workloads, suitable for large-scale data operations.
- Supports custom UDFs and integration with other Hadoop ecosystem tools.
Cons
- Does not support online transaction processing (OLTP); focused on OLAP instead.
- Subqueries are not supported, which can limit query complexity.
- High query latency, making it less suitable for real-time data querying.
- Lacks support for transaction processing features.
- Primarily supports non-real-time or "cold" data querying, not suited for real-time analytics.
What next?
"If you're tired of the high costs and complexity of Google BigQuery, exploring these google bigquery alternatives might be time to switch to something simpler and more cost-effective.
Moving away from BigQuery to a platform that combines all your data needs—like ingestion, storage, transformation, and business insights—can save you time and money.
That’s what 5X brings to the table. 5X is designed to be easy to use and quick to set up. It’s a one-stop platform that handles everything from start to finish, so you don’t have to worry about dealing with multiple tools or high costs.
You can get everything you need up and running in just hours, and save up to 30% on your data expenses.
Plus, it frees you up to action data so you don’t have to worry about the underlying tools & integrations.
FAQs
Is BigQuery open source?
How does Amazon Redshift compare to Google BigQuery?
Is Google BigQuery free?
Building a data platform doesn’t have to be hectic. Spending over four months and 20% dev time just to set up your data platform is ridiculous. Make 5X your data partner with faster setups, lower upfront costs, and 0% dev time. Let your data engineering team focus on actioning insights, not building infrastructure ;)
Book a free consultationHere are some next steps you can take:
- Want to see it in action? Request a free demo.
- Want more guidance on using Preset via 5X? Explore our Help Docs.
- Ready to consolidate your data pipeline? Chat with us now.
Get notified when a new article is released
Cut GBQ costs—switch to 5X
Cut GBQ costs—switch to 5X
How retail leaders unlock hidden profits and 10% margins
Retailers are sitting on untapped profit opportunities—through pricing, inventory, and procurement. Find out how to uncover these hidden gains in our free webinar.
Save your spot
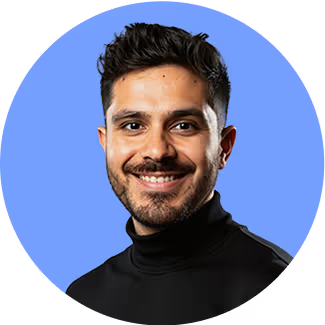