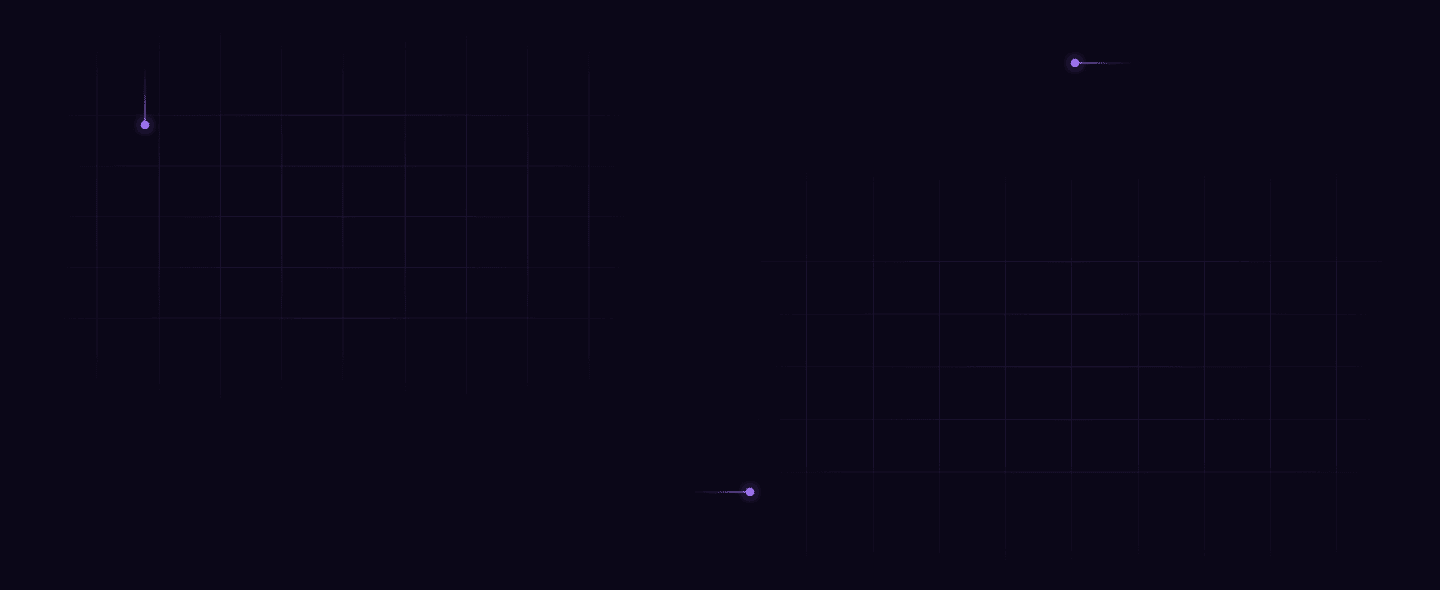
Every month, your sales team throws in their forecasts (gut feeling, a few Excel sheets, some CRM data, and maybe a whisper of experience). Sometimes, they nail it. Other times it’s a shot in the dark.
You know something’s off. Deals stall. Revenue projections swing wildly. Your board asks why targets weren’t met, and all you have are best guesses.
Meanwhile, your competitors, especially the new ones, seem to predict their pipeline with eerie accuracy. They adjust pricing in real time. They know exactly when a deal is about to go cold.
How? They’re using predictive sales analysis.
And you can too! You already have all the data you need. It’s just buried across spreadsheets, CRMs, emails, and sales calls. But without the right tools, it’s as good as invisible.
So let’s change that. Let’s talk about how predictive sales analysis can turn your existing data into a competitive advantage without hiring an army of data scientists.
What is predictive sales analysis?
Predictive sales analysis is the art (and science) of using historical data, AI, and machine learning to forecast future sales with high accuracy.
It analyzes past deals, customer behavior, market trends, and even external factors (like seasonality or economic shifts) to tell you:
- Which deals will close and when
- Which customers are ready to buy again
- Which reps are on track (or falling behind)
- Where to focus efforts for maximum revenue
Instead of relying on instinct, predictive sales analysis gives you data-backed insights that reduce uncertainty and improve decision-making.
Why is predictive sales analytics important?
Right now, your sales team is probably making decisions based on experience, intuition, and a few scattered reports. Sometimes, they get it right. But when they don't, it costs you:
- Missed quotas. Reps chase the wrong leads, and deals slip through the cracks
- Inaccurate forecasts. You overhire or underhire, misallocate budgets, or miss growth opportunities
- Lost revenue. You leave money on the table by not upselling or cross-selling at the right time
- Frustrated customers. Sales outreach feels random instead of personalized and timely
With predictive sales analytics, things take a complete 180-degree turn.
In fact, companies that use predictive analytics are 2.9 times more likely to report revenue growth at a rate higher than the industry average.
Here's what changes with predictive sales analysis:
- Sales teams focus on the right deals. AI identifies high-probability leads, so reps spend time where it matters
- Revenue forecasts become accurate. You can plan hiring, budgets, and resources with confidence
- Pipeline risks get flagged early. You know which deals are in danger and can act before they die
- Customer retention improves. Predictive insights show when a customer is likely to churn—giving you time to intervene

However, predictive analytics is only as good as the data it runs on. If information is siloed across different platforms, forecasts become incomplete or misleading. That’s why companies prioritize data consolidation, ensuring a unified, AI-ready dataset for more precise predictions.
"Once you have all that interaction data, you can marry it with the CRM data and start figuring out the probability of closing each of the sales opportunities. How much is it going to close for? When will it close?"
— Michael Tambe, Sr. Director, Data & Analytics, Gong
Improving Gong’s customer retention with predictive data analytics
Traditional forecasting vs. Predictive sales forecasting
Traditional sales forecasting relies on past sales data, gut instinct, and manual inputs from sales teams. It assumes that historical trends will repeat, making it prone to human bias and inaccurate estimates. Since it doesn’t adapt to real-time changes, businesses often miss warning signs like shifting customer behavior or market disruptions.
Predictive sales forecasting, on the other hand, uses AI and machine learning to analyze historical data alongside real-time inputs like customer interactions, market trends, and external factors. It continuously learns and adapts, providing more accurate, data-driven insights. This proactive approach helps businesses identify risks early, optimize sales strategies, and make smarter, faster decisions.
This is why 5X is built to pull structured and unstructured data from your CRMs, emails, and external sources without disrupting your existing workflows.
6 Benefits of predictive sales forecasting
Predictive sales forecasting helps you make smarter decisions, achieve higher efficiency, and stay ahead of the competition. Let’s see how:

#1 More accurate revenue predictions
AI-driven insights reduce human error and provide data-backed forecasts instead of gut-based guesses. Unlike traditional forecasting, which often misses sudden market shifts, predictive models incorporate external factors like economic changes, seasonality, and competitor activity.
For example, a SaaS company using AI-driven sales forecasts can anticipate subscription renewals, identify upsell opportunities, and reduce churn.
#2 Better decision-making
With real-time data, sales leaders can adjust strategies on the fly, allocate resources efficiently, and avoid over or under-hiring. Instead of relying on outdated quarterly reports, predictive analytics provides continuous insights, helping businesses respond instantly to market changes.
For instance, a retail company using predictive forecasting may adjust its inventory and marketing spend based on AI-generated demand insights.
#3 Higher sales team productivity
Predictive forecasting prioritizes the most promising leads, allowing reps to focus on deals most likely to close—boosting conversion rates. AI can analyze customer intent, past interactions, and buying signals to tell sales teams who to call, when, and what to pitch.
#4 Early risk detection
It flags at-risk deals before they fall apart, giving teams time to course-correct instead of reacting too late. Predictive models analyze factors like stalled email responses, pricing objections, and competitor involvement to assess deal health.
Example: Say, a manufacturing firm detects patterns indicating a 30% likelihood of contract loss for key accounts. By acting early, they can renegotiate contracts and retain over $2M in potential lost revenue.
#5 Improved customer retention
By analyzing buying patterns, AI predicts when customers are likely to renew, upgrade, or churn, enabling proactive engagement. Sales teams can reach out before a contract ends or offer targeted discounts based on AI-driven insights.
#6 Competitive edge
AI-powered forecasting enables dynamic pricing strategies, better demand forecasting, and smarter go-to-market plans.
How to implement predictive sales analysis
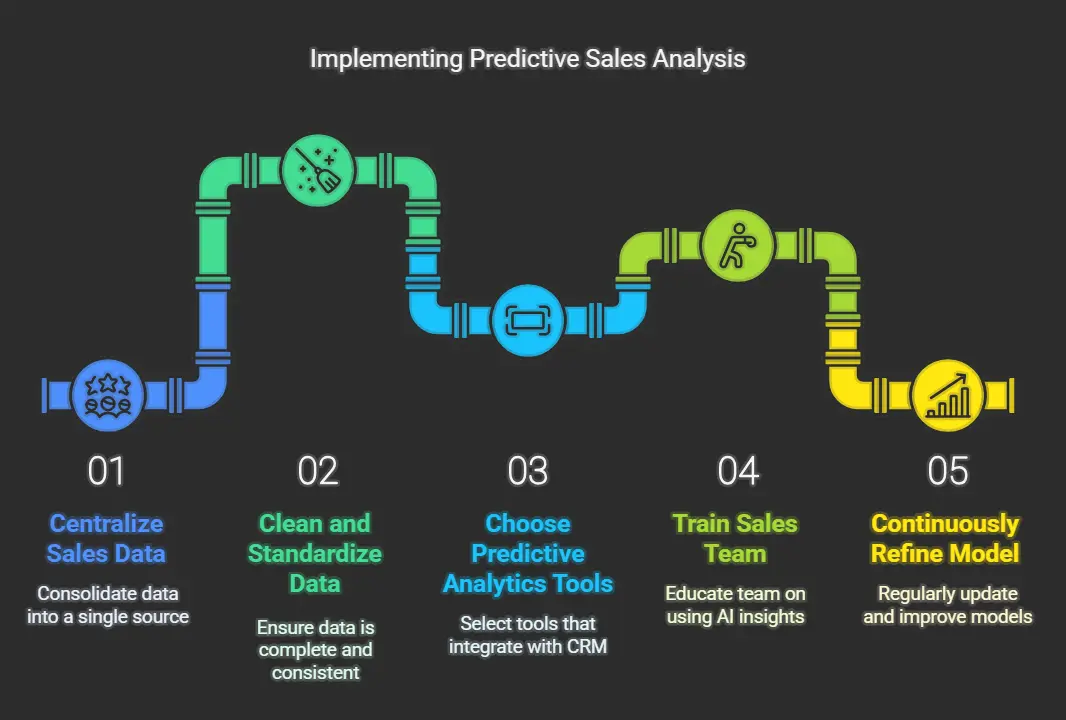
Implementing predictive analysis software doesn’t require a PhD in data science. You just need the right approach, tools, and mindset. Here’s how to get started:
#1 Centralize your sales data
Your data is likely scattered across CRM systems, spreadsheets, emails, and call logs. But to make predictive analytics work, you must first ensure you can pull data from various sources (CRMs, emails, and third-party tools) into a structured format and consolidate it into a single source of truth. This is where data ingestion tools play a critical role in harmonizing and preparing data for AI-driven forecasting.
#2 Clean and standardize data
Messy, inconsistent data leads to inaccurate predictions. Ensure your data is complete, up-to-date, and standardized before feeding it into an AI model. This includes:
- Removing duplicate or outdated records
- Standardizing lead scoring criteria
- Ensuring CRM entries are correctly formatted
#3 Choose the right predictive analytics tools
You don’t need to build AI models from scratch. Many sales platforms, like Salesforce Einstein, BoostUp, Clari, and Gong, offer built-in predictive analytics that integrate with existing CRMs. Look for tools that:
- Provide real-time deal insights
- Offer AI-driven lead scoring
- Identify pipeline risks early
#4 Train your sales team to leverage AI insights
AI is only as useful as the people using it. Sales teams need to trust and understand predictive insights—so make training a priority. Show them:
- How AI ranks leads and opportunities
- Why certain deals are at risk
- How to act on predictive recommendations
#5 Continuously refine and improve your model
Predictive analytics is an evolving system. Regularly update your models with new data, adjust based on feedback, and test different variables to improve accuracy.
"If you can just ask the [Gen AI] model, 'Hey, why did this account churn? It had great usage,' or 'Hey, why was this account retained? It had horrible usage,' you can quickly get to that level of generating new hypotheses, getting into the data, and making it work. And that’s when you will start to see AI and your company come together.”
— Michael Tambe, Sr. Director, Data & Analytics, Gong
Improving Gong’s customer retention with predictive data analytics
5 Challenges of predictive analytics in sales forecasting
Predictive analytics can transform sales forecasting, but it’s not without obstacles. Here are some common challenges businesses face and how to tackle them:
#1 Data quality issues
Your predictions are only as good as your data. Incomplete, outdated, or inconsistent data leads to inaccurate forecasts. Many businesses struggle with duplicate CRM entries, missing fields, or unstructured data from emails and calls.
Solution: Regularly clean and standardize your data. Use Master Data Management (MDM) tools to maintain a single source of truth across all systems.
#2 Resistance to AI-driven insights
Sales teams often distrust AI recommendations especially if they contradict human intuition. If reps don’t trust the system, they won’t use it.
Solution: Train sales teams on how AI works and show real-world examples of its accuracy. Start with small wins to build confidence.
#3 Integration with existing tools
Many companies use multiple CRMs, spreadsheets, and sales platforms, making it hard to consolidate data for predictive analysis.
Solution: Choose predictive analytics tools that integrate seamlessly with your existing CRM (Salesforce, HubSpot, Microsoft Dynamics, etc.).
#4 Difficulty in adapting to real-time market changes
Traditional models may not react fast enough to sudden market shifts, competitor moves, or economic changes. Static predictions can become unreliable.
Solution: Use real-time AI-driven forecasting models that adjust dynamically based on new data inputs.
#5 High initial investment and complexity
Some companies hesitate due to the cost of AI-powered tools or the perceived complexity of implementation.
Solution: Start small. Leverage pre-built AI solutions like Clari, BoostUp, or Gong, which integrate easily and offer fast ROI.
Start in 48 hours!
Traditional businesses, whether in retail, banking, manufacturing, healthcare, or real estate, can’t afford to wait for AI to "someday" transform sales forecasting. The technology is already here, but the real challenge isn’t AI itself; it’s accessing and preparing the data.
Your legacy systems (SAP, Salesforce, Oracle, and more) hold the key to predictive sales insights, but without a structured approach to data extraction, centralization, and cleaning, AI-driven forecasting remains out of reach. That’s why businesses need a solution that doesn’t just promise AI-powered results but actually bridges the gap between traditional data and AI implementation. This is basically what 5X does.
You don’t have to wait months to see results. With 5X’s 48-hour jumpstart, we take your existing sales data, structure it, and connect it to AI-driven predictive analytics giving you real-time sales forecasting without the complexity.
Which algorithm is best for sales prediction?
How to calculate predictive sales?
How are sales forecasts determined?
How does predictive analytics work?
Building a data platform doesn’t have to be hectic. Spending over four months and 20% dev time just to set up your data platform is ridiculous. Make 5X your data partner with faster setups, lower upfront costs, and 0% dev time. Let your data engineering team focus on actioning insights, not building infrastructure ;)
Book a free consultationHere are some next steps you can take:
- Want to see it in action? Request a free demo.
- Want more guidance on using Preset via 5X? Explore our Help Docs.
- Ready to consolidate your data pipeline? Chat with us now.
Table of Contents
Get notified when a new article is released
Get an end-to-end use case built in 48 hours
Get an end-to-end use case built in 48 hours
How retail leaders unlock hidden profits and 10% margins
Retailers are sitting on untapped profit opportunities—through pricing, inventory, and procurement. Find out how to uncover these hidden gains in our free webinar.
Save your spot
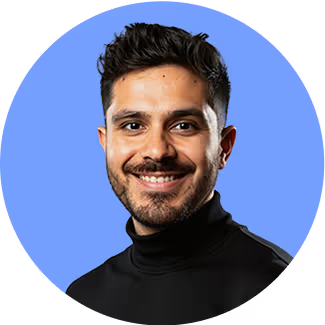