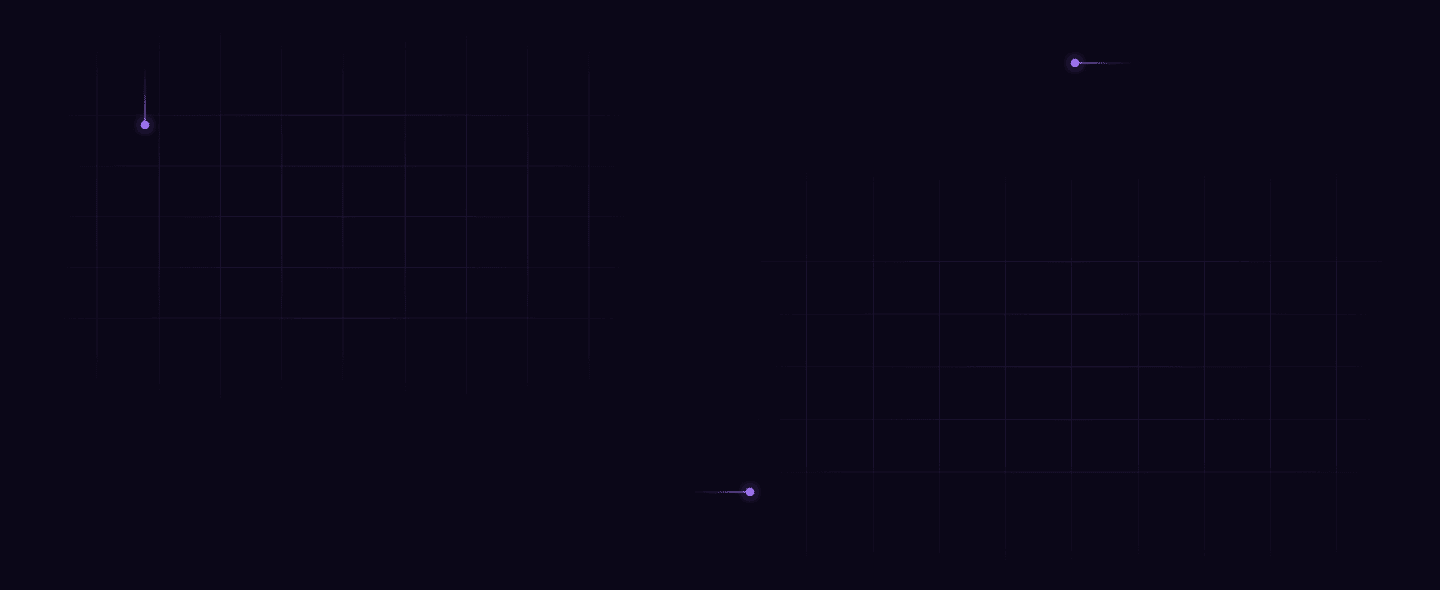
Rise of the Data Generalist: Smaller Teams, Bigger Impact
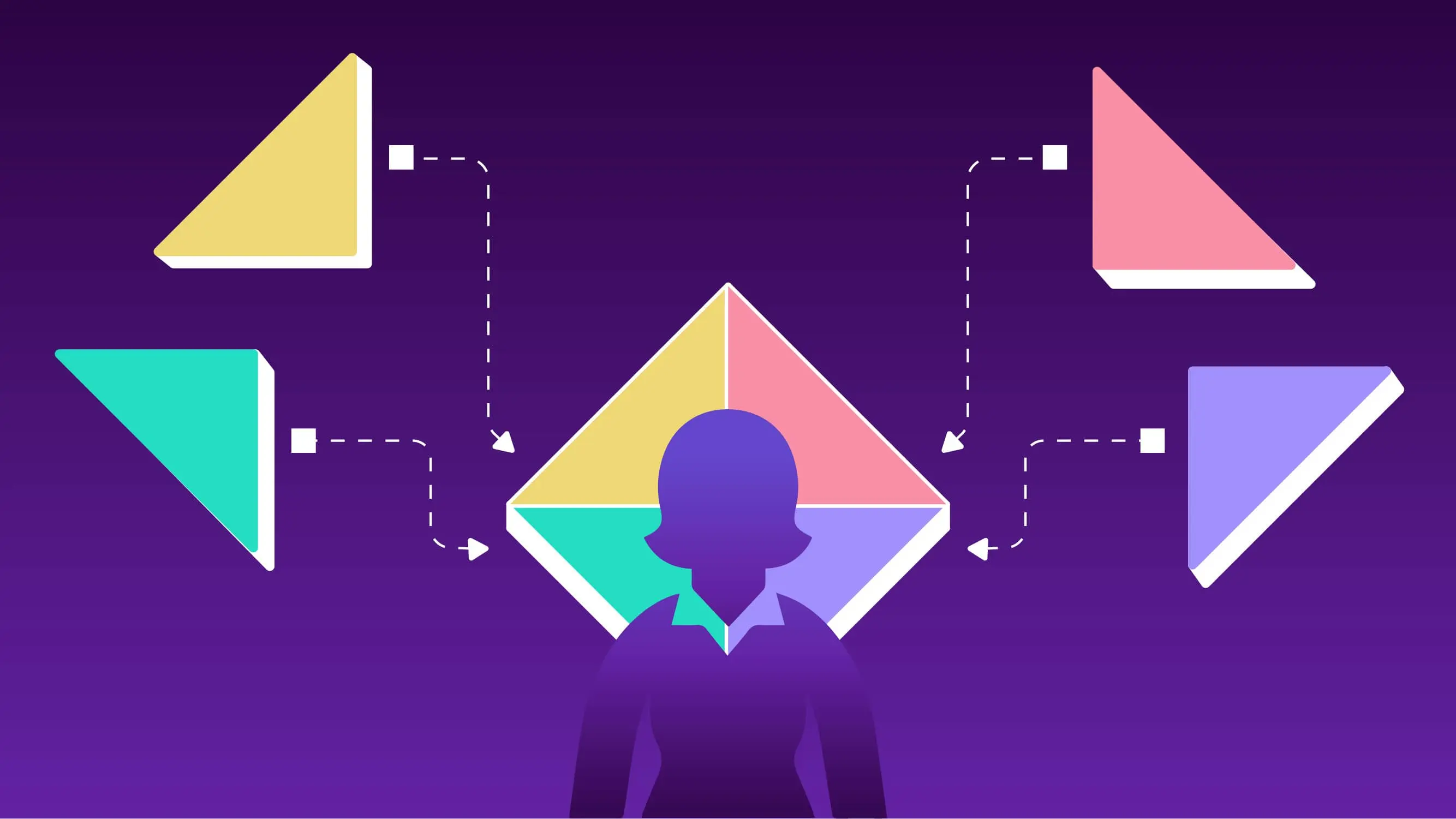
In the evolving data landscape, driven by AI and advanced data vendors, data teams are expected to become more compact, prioritizing efficiency over team size.
In a landmark blog post in 2017, Maxime Beauchemin talked about the "Rise of the Data Engineer." This marked the moment when data engineering became a recognized job title, with Facebook leading the way in 2012. But let's take a step back and see how we got here.
Looking back: Data boom and specialization
A decade ago, we saw the rise of cloud-based super apps like Facebook, Yelp, and Foursquare. These apps needed to handle vast amounts of data in the cloud, and we didn't have the right tools and infrastructure to make the most of this data. Things like data pipelines (ways to move data), data storage, modeling (making data usable), business intelligence (BI), and other tools were either not around or just getting started. This deficiency in data management tools led to the need for specialization in the field.
The changing face of data roles
A new breed of professionals, known as "data engineers," emerged in response to this growing challenge. They were responsible for creating tools and infrastructure to handle and optimize data in the cloud, filling the crucial gap.
Over the past decade, as the data landscape has evolved, we've witnessed the emergence and transformation of various roles within the field to meet the changing demands of the data ecosystem.
1. Data Analysts: They translate structured, modeled data into actionable business insights. They play a vital role in interpreting data effectively, empowering organizations to make informed decisions.
2. Analytics Engineers: Building upon the foundation of data analysis, analytics engineers, often associated with dbt, introduced software engineering practices to the data world. Their focus shifted towards building analytical models, reducing the need for ad hoc analysis, and progressively converging with data engineering responsibilities.
3. Data Scientists: The need for data scientists arose with the increased availability of structured and actionable data. These individuals possess expertise in statistics, programming, and domain knowledge, allowing them to extract valuable insights from extensive and intricate datasets. Their role involves informing decision-makers and uncovering data-driven patterns to shape business strategies.
4. Data Visualization Engineers: Specializing in transforming raw data into easily understandable visual representations, data visualization engineers combine data analysis, design, and technical skills. Their objective is to create visually appealing graphics that facilitate interpreting complex information.
But here's another reason we ended up with so many roles: the data world got really complicated. Each part of the data puzzle had its own set of tools. This complexity meant companies needed bigger teams to manage it all, translating to larger spends and management challenges.
This trend was fueled by buzzwords like 'data is the new oil' and cheered on by investors and pundits. As a result, companies often boasted about the size of their data teams.
However, 18 months ago, things took a turn...
Achieving more with less
It's time to refocus on the true purpose of these teams: driving business growth. It's no longer about building large teams or using trendy tools; it's about generating ROI for the business. The era of unchecked spending on data teams is behind us. Today, our goal is efficiency, which is going to be achieved by solving for both the platform and people:
Reducing the overhead of managing multiple vendors:
As the data landscape becomes increasingly complex, "Fully Managed" is the newest category on the block. It eliminates the need for managing multiple vendors, handling vendor discovery, POCs, negotiation, procurement, integration, and maintenance.
There are over 500 vendors in 30 different data categories. The analogy we use is all these vendors are selling car parts. Imagine walking into a Honda and instead of selling you a Civic, they sold you an engine and you had to build your own car. Businesses spend a lot of time figuring out which vendors to use and how to integrate them. This results in large, cumbersome data teams to manage these vendors, leading to rising costs and complexity.
The fully managed category will give you all of the advantages of an end to end platform with the flexibility of best in breed vendors. Depending on your industry, use case, size and budget you will be able to build a tailored platform for your business.
By 2025, I anticipate that 30% of data teams will migrate to a fully managed solution.
Creating a lean team that moves fast:
As we start to consolidate tooling, we have an equally or arguably bigger opportunity to reduce team size; “Do more with less” is becoming a theme. People costs represent a substantial portion of a company's expenses, making this shift significant. Moreover, tooling has become more mature, and many current data roles would not justify dedicated titles moving forward. As a result, we're witnessing the rise of a new archetype—the "data generalist"—who can operate around different areas of the data stack. Here are a few examples of consolidation in titles in light of this shift:
1. Data platform engineers - They typically comprise 20% of the data team. Tooling consolidation and full managed solutions will allow them to adeptly manage complex tasks like vendor integrations, access control, governance, and security, often without dedicated resources.
2. Data engineers - The rise of data generalists will enable them to utilize automated ingestion tools to construct and manage data pipelines with increased efficiency.
3. Data analysts / Analytics engineers -The need for specialized analytics engineers and data analysts is on the decline. Since intuitive tools and concepts, like activity schema, have made data modeling simpler and more accessible. Moreover, some of these tools offer helpful insights and recommendations, making it easier to take on these tasks.
4. Data scientists - We're going to see a lot of AI platforms that run complex models. These platforms require little knowledge to tweak and feed your data in. This will pave the way for generalists to operate at a higher level with less sophistication
5. BI engineers - The rise of conversational BI on top of the semantic layer has automated the tasks usually executed by BI Engineers. Additionally, LLM features like chat have made it far more intuitive to answer business questions.
Sure, we will still need specialized talent, especially at larger companies, and will still have enough workflows for each role, but in general, smaller to medium-sized companies would need their data team members to have generalist skills as well. Over time, there is a possibility that the next generation focuses on an all-rounded approach to data instead of specializing in data science, data engineering, or other skill sets. Existing specialists will also need to retrain to gather more rounded expertise.
Specialists who don't retrain run the risk of getting left behind. For example, a number of years ago, Microsoft stack specialists worried their skills had limited use outside Microsoft as the industry. Just like everything else, highly skilled specialists will continue to excel, but an increasing percentage may struggle to adapt to the job market, which starts to favor generalists in an environment with a shrinking number of highly specialized roles.
In conclusion
The data landscape has evolved significantly. We've moved from having big teams to focusing on efficiency.
This shift has given rise to data generalists who can handle various tasks, making traditional roles less necessary. Larger teams may still specialize, but the skills gap is widening, prompting specialists to explore other paths.
Adaptability is key. Expect lean, agile, and highly effective data teams building on top of fully managed data platforms.
Building a data platform doesn’t have to be hectic. Spending over four months and 20% dev time just to set up your data platform is ridiculous. Make 5X your data partner with faster setups, lower upfront costs, and 0% dev time. Let your data engineering team focus on actioning insights, not building infrastructure ;)
Book a free consultationHere are some next steps you can take:
- Want to see it in action? Request a free demo.
- Want more guidance on using Preset via 5X? Explore our Help Docs.
- Ready to consolidate your data pipeline? Chat with us now.
Wait!
Don't you want to learn how to quickly spot high-yield opportunities?
Discover MoonPay’s method to identify and prioritize the best ideas. Get their framework in our free webinar.
Save your spot

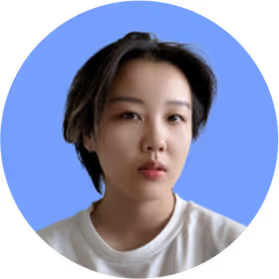