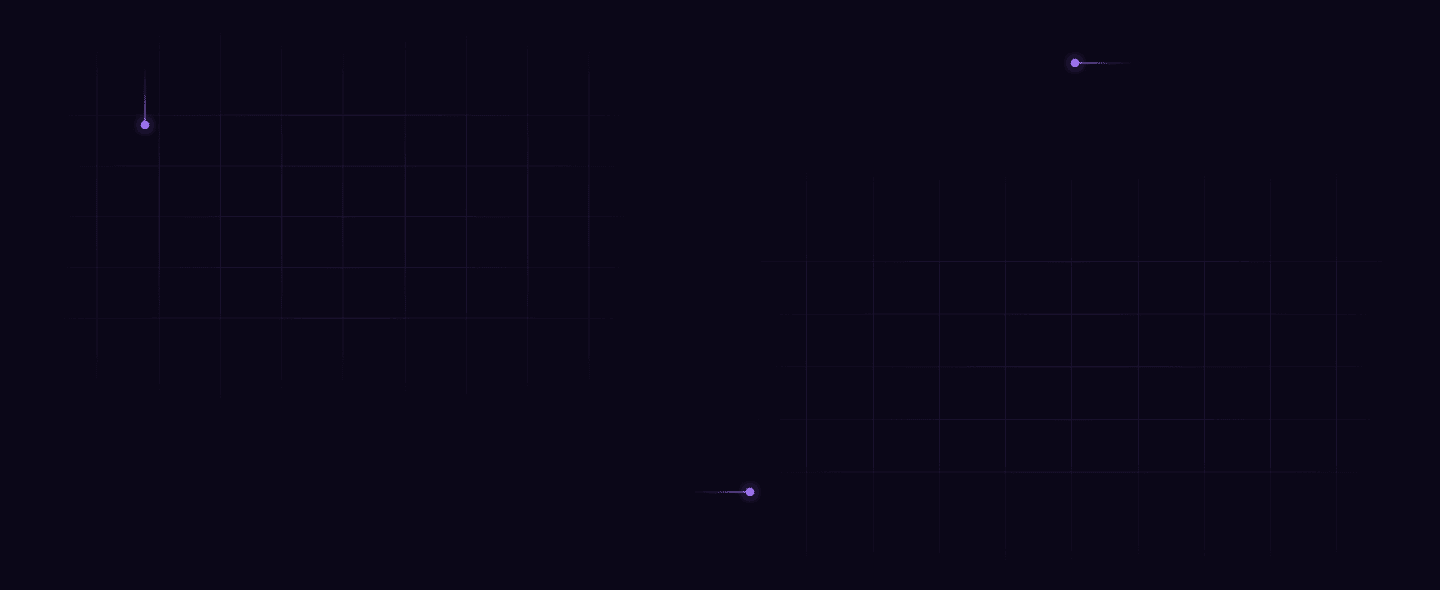
Friends Don’t Let Friends Build a Data Platform
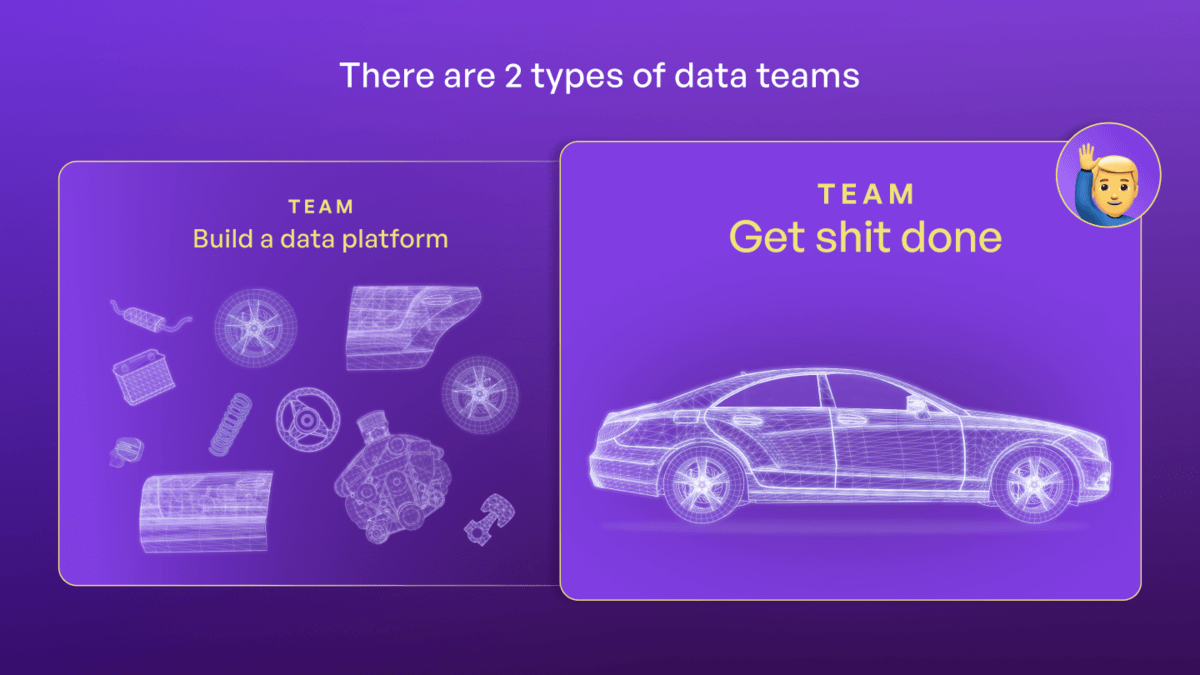
When I left WeWork in 2020 to start 5X, it was after years of seeing the same data challenges across industries. My journey began as one of the first data engineers at Salesforce, back then none of the data infra layers existed. Many years later at WeWork, we managed vast amounts of data - not just pixels but also bricks. We needed to be early adopters of many tools - dealing with over 100 different data sources.
There was no choice but to use multiple specialized vendors because no one else had the capabilities we needed. By the end of it we had a 100+ data org and out of that 20 people were managing our data platform. That was over a 4m/year cost center on building a platform that wasn’t differentiated in any way from the next company.
The primary challenge with the fragmented ecosystem is that for the vast majority of companies, flexibility comes at a cost of resources, cost and efficiency. A trade off most companies don't need to make.
What is a full stack data platform?
Today we have 500+ vendors in 30+ categories. The analogy we use is that the majority of vendors today are selling car parts. Imagine walking into a Honda and instead of selling you a “Civic” they sell you an engine and you have to build your own car.
We would most definitely see a lot fewer cars on the street. That's because building and maintaining a car is real work and customers just want to get from place A to place B.
In this analogy a full stack data platform is a car you can drive off the lot.
A full stack data platform combines all of the components you need to ingest, centralize, model and report on data. It integrates various stages of data management—from collection to analysis—into one system. Other categories can be added on top of a full stack data platform but full stack data platforms alone are capable of building end to end data use cases on top of.
A true data readiness platform integrates these main components:
- Data ingestion: Automated ingestion from various sources into a data lake/warehouse. The average company today has about 30+ data sources.
- Data storage: Centrally store your data in a semi structured manner for analysis
- Data compute: Bring a compute layer on top of this storage layer
- Data transformation: Clean, structure and model your data for analysis. This is one of the core layers. The resultant data set is consumed not just for analysis and BI but also becomes the foundation for AI and data activation (push this data back to source systems where stakeholders can consume it in tools they already use)
- Data orchestration: Automating the data flow and processing. As your number of data sources and models increase we end up with complex DAGS which need more than simple cron jobs to execute.
- Business Intelligence: Turning data into actionable insights through dashboards and reports
Most of the vendors you see in the data space focus on just a single or a few components over here and you need to use multiple vendors in the ecosystem to build a full stack data platform.
Full stack data platforms replace “Build your own platform” for 90%+ of customers
Moving back to the car part analogy. The only people who would want to build their own car are racing teams or enthusiasts. For the vast majority of people buying a stock car would do and far another group of people buying a stock car and then tuning it up would make sense. The % of people who would build a car from scratch would be very small.
In the same way unless data is your product or you’re doing something extremely custom it makes no sense to build a platform from scratch. For the majority of use cases, it will be faster, cheaper and easier to use an all in one data platform.
While all in one data platforms are relatively new compared to single category solutions these are quickly maturing and will catch up on larger enterprise use cases like lineage, catalog, data activation and governance. Another option is to use them for your core data layers and choose to work with separate vendors for some of the use cases around lineage, governance and catalog similar to buying a stock car and tuning it up for your use cases.
The advent of full-stack data platforms marked a turning point. These platforms integrated all aspects of data management into a single, cohesive system.
Julien Hurault, in his recent article on Data Stack Rebundling, says, “The rise of full stack data platforms as indicative that the ecosystem is maturing progressively and entering its democratization phase now.”
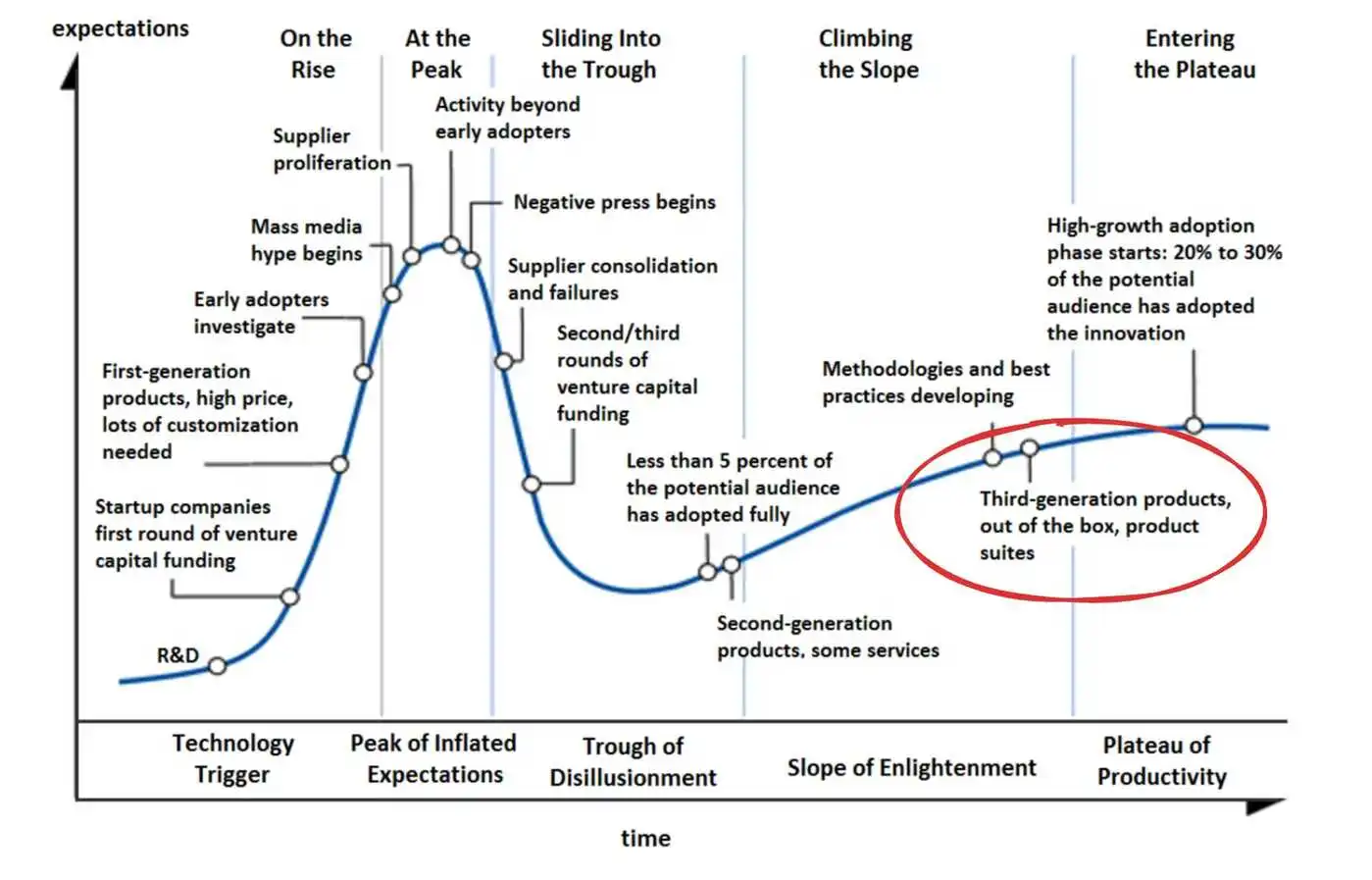
Why full stack data platforms are the future
The transformation from fragmented data systems to full-stack data platforms marks a significant shift in how businesses handle data. That’s why they are set to shape the future of data management.
1. Everything is moving to AI
AI is fundamentally changing businesses. Every new company is going to be an AI company. By now, we all know that you can't have an AI strategy unless you have a data strategy and if you dont understand your data, neither will AI.
The reality is that AI is going to have its own stack on top of your platform just like your data and analytics suite has its own stack. Some of the components will converge (workflows, storage and compute layers) but they will be specific tooling at the AI layer as well.
If we're going to have additional tooling at the AI layer, we can't afford to have 5-7 tools at the data & analytics layer. Abstraction always moves upstream and its time to consolidate the data layer to make room for AI.
2. You spend zero time on vendor discovery and management
One of the pain points with fragmented data systems is the need to constantly integrate different tools, each handling a specific part of the data lifecycle. Its not just about integrating tools - vendor discovery, procurement, management, training and communication all require resources. Sure going for vendor sponsored lunches and parties can be fun but doesn't that take away from actually using data to generate an ROI for the business.
Full-stack data platforms eliminate these integration headaches by providing a unified ecosystem where all data processes—from ingestion to visualization—work seamlessly together. This simplifies data management but AND reduces the risk of errors and inconsistencies.
Procurement is another area which depends on multiple teams - Legal, Finance, Security groups are typically involved and each vendor needs to go through the same lengthy process. These are typically invisible costs as they exist outside of the technology budget but are absorbed by the business in other areas. Ultimately they affect operating costs of the business.
3. You get end to end workflows
Traditional data systems, with their fragmented nature, often have compartmentalization in their workflows.
An example is ingestion might be handled by an automated ingestion layer. The modeling layer does not have access to this information and has to rely on the data being there. And the same story for the BI Layer. This causes disjoint workflows and isn't a great developer experience.
By using a full stack data platform we can stitch all of the components together into a cohesive workflow so you can build end to end workflows.
Another example we see is companies using dbt cloud of enterprise. dbt is a great tool but the cloud version comes with its own orchestrator. This is troublesome because the dbt orchestrator is quite simple and doesn't support advanced DAGS. This leads to many of these companies also having an Airflow or Dagster orchestrator for more advanced use cases. This means that they are now multiple different orchestrators and that exponentially increases complexity. One workflow is on one tool and another is on the other. If you want to combine them or build a DAG across them, it requires a large rewrite.
With full-stack data platforms, this problem addresses itself. We’re able to deploy a central workflow across all of the different components.

4. Your total cost of ownership (TCO) decreases significantly
We have seen that all in one platforms have a ~30% lower TCO as compared to custom platform build outs. This only takes into account the cost of the tooling (not the cost of the build out and maintenance - which we will get to)
This makes sense when you take into account each of the vendors you work with need to maintain their own sales, marketing, customer success, support teams. All of those costs ultimately get passed back to the customer.
The second factor to consider over here is the cost of build out & ongoing maintenance. This is typically done by data platform teams.
If we are to look at the size of data teams they are typically 20% of the size of your entire data team. For example, at WeWork, when we ran a 100 person data group we had about 20 people in our data platform team.
The data platform team is responsible for vendor discovery, selection, integration, access control, security and governance. All of these are critical when you build a custom platform but get standardized to a large extent in an all in one platform.
The cost of building a data platform ultimately is the nail in the coffin. In WeWork’s example, a 20-person data platform team cost us $4 million dollars/year, which was 2x our data infrastructure cost at the time.
Moving to an all in one data platform would have saved us between 200-250% of the cost we incurred building our own custom data platform.
5. You see an uptick in productivity
Context switch is a real thing. It doesn't apply just to switching between different tasks it applies to data teams as well.
Custom build out means you have multiple tools in your setup. This means a data engineer needs to switch between multiple tools on a daily basis
- Data ingestion tool - for managing pipelines
- Warehouse - for querying data
- Data modeling tool - Building and testing data models
- Data Orchestration - Scheduling jobs and DAGS
- Data observability - Data quality
- BI - reports and dashboards
That is a lot of context switching for a single function (Data). Given where we are going in AI a lot of this context switching is needed to get to data readiness and post this the AI world is going to have its own set of tools. The numbers just don't add up.
Having a single platform for data readiness typically makes your data teams 10-15% more productive on a daily basis.
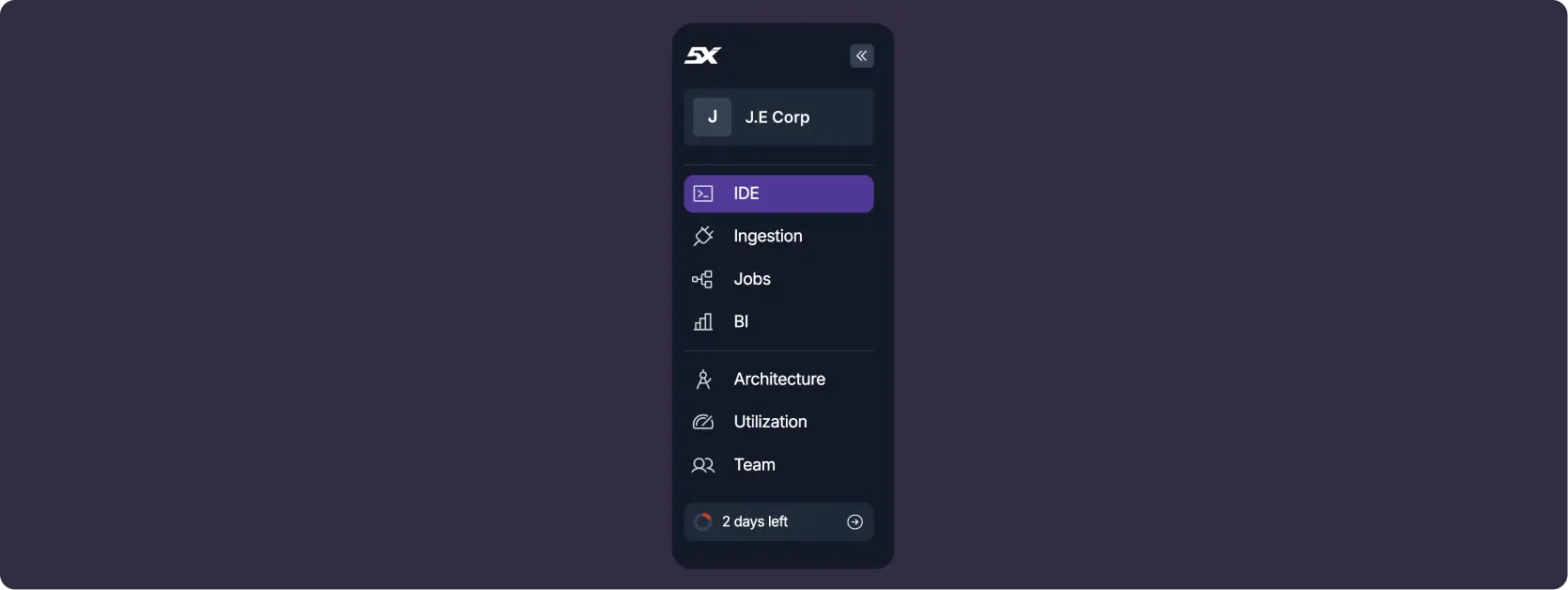
6. Your data remains safe
Data security and regulatory compliance are critical concerns for any business handling sensitive information. Single category vendors' systems are not less secure. The problem arises when you manage multiple vendors and the interoperability between them becomes a risk factor.
You need to constantly make sure that the interfaces between them are secure. That means rotating keys every 6 months, checking for updates in vendor access management and occasional Pen tests to make sure your data platform is secure.
The weak link often becomes data governance since it often is controlled by people teams. Making sure that everyone in the company has the correct access is challenging as is but to do it across multiple tools makes this process more complicated.
Full-stack data platforms address this by providing robust security features such as encryption, access controls, and auditing capabilities built into the system. This integrated approach ensures that data is protected and compliance with industry regulations is maintained without additional overhead. This is especially important as data privacy regulations become more stringent.
So, when do you know it's time for a full-stack data platform?
1. When your data team is constantly looking for new vendors
This is a tell tale sign of thinking a new vendor is going to solve all your problems. The incentives of your data platform team are not aligned with business incentives. Every new tool requires weeks of setup and constant troubleshooting, you’re losing valuable time and resources.
2. When overall cost of ownership is becoming a concern
As we mentioned earlier we see all in one platforms decrease infra costs by ~30% and more importantly overall data Costs by over 100% when you take into account people teams needed to maintain the data platform. Moving to an all in one platform is the single best decision you can make to decrease TCO quickly and permanently.
3. When your data team spend too much time focusing on data pipelines
Building data pipelines have been replaced by automated data ingestion. Not only do we have 600+ pre-built connectors but we are also able to build custom connectors for our customers in hours or days. No more time spent on connectors and more time on analysis
4. When you need quick support and guidance
Multiple vendor strategies means you are left to your own when it comes to support. Each component is only looking at supporting their individual area. No one is looking at this holistically
All in one platforms have the advantage of providing support end to end. You don't need to care if something is wrong in your model or your DAG is failing or is it a data pipeline issue. Premium end to end support is able to look at all of these issues holistically.
5X is also the only all in one platform which runs a full service data consultancy. What this means is we are able to provide long term resources or come in and do project based implementations to help you get started. Data platform + Data services = Data as a service.
The road ahead
The future of data management is all about simplicity and speed. Full-stack data platforms are leading this change, making it easier for businesses to handle their data efficiently. Gone are the days of juggling multiple tools and dealing with constant integration issues. Instead, everything you need is in one place, working seamlessly together.
This shift means businesses can get insights faster, make better decisions in real-time, and save money by not having to pay for multiple systems. The industry is moving towards these unified platforms because they help companies grow without getting bogged down by data problems.
At 5X, we've built our platform to meet these needs. We focus on making your data journey smooth and cost-effective, with all the tools you need in one package. Whether you’re looking to streamline your operations or get quicker insights, 5X is designed to help you achieve that.
Perhaps if this existed in 2018, companies like WeWork would not have needed to spend $4m/year in building a data platform. In any case, after reading this, neither should you.
All in one platforms are going to quickly catch up and overtake custom builds.
And don’t take my word for it, try 5X for free. If you’re ever interested in chatting about the data space I would love to connect. You can reach me at tarush@5x.co
Building a data platform doesn’t have to be hectic. Spending over four months and 20% dev time just to set up your data platform is ridiculous. Make 5X your data partner with faster setups, lower upfront costs, and 0% dev time. Let your data engineering team focus on actioning insights, not building infrastructure ;)
Book a free consultationHere are some next steps you can take:
- Want to see it in action? Request a free demo.
- Want more guidance on using Preset via 5X? Explore our Help Docs.
- Ready to consolidate your data pipeline? Chat with us now.
Wait!
Don't you want to learn how to quickly spot high-yield opportunities?
Discover MoonPay’s method to identify and prioritize the best ideas. Get their framework in our free webinar.
Save your spot

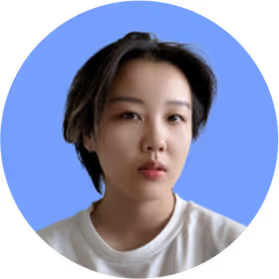