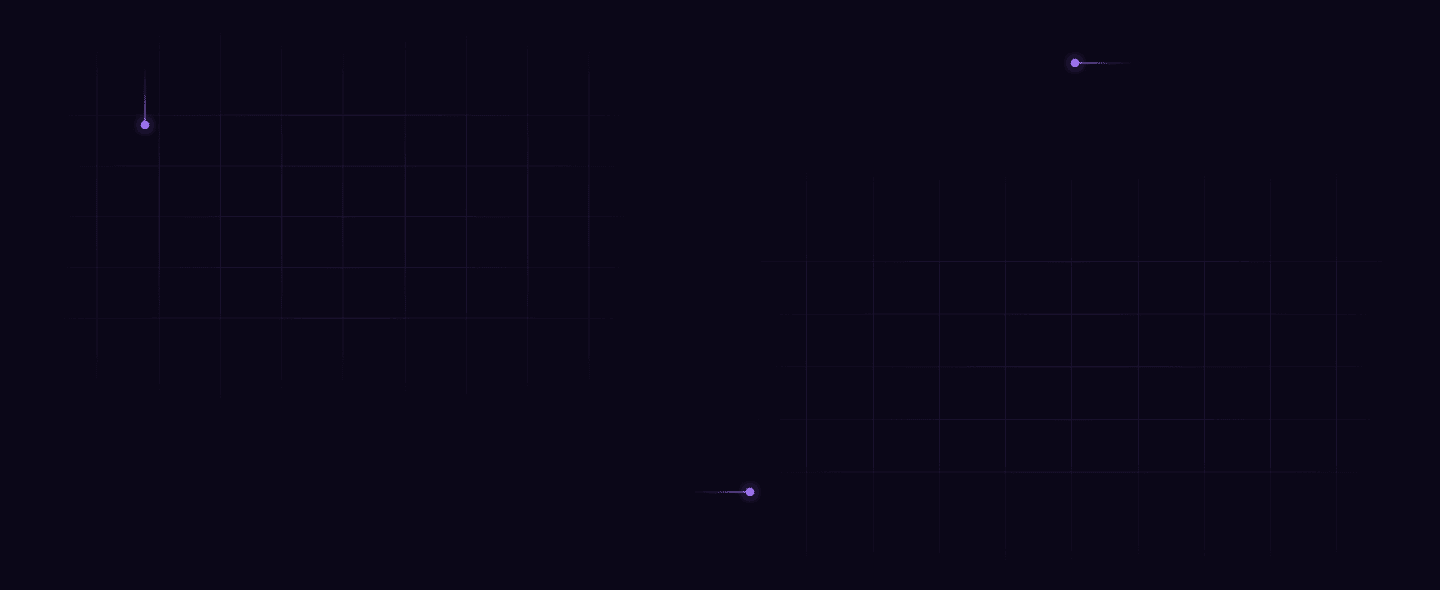
Data interpolation is a mathematical technique used to estimate unknown values between two known data points. It helps "fill in the blanks" where data is missing, offering an informed estimate based on the pattern of existing data. This method is used in a wide range of fields. For example: statistics, economics and business intelligence. The process of data interpolation helps analysts make informed decisions even when complete data sets aren’t available.
Consider a scenario where a company tracks sales data every quarter, but some values for certain months are missing. Interpolating data allows you to estimate the missing values based on the existing data trend. While it's not about generating new information, it helps complete the dataset, allowing for more accurate analysis.
In modern data platforms, interpolation is critical in ensuring data quality. Tools like 5X simplify data ingestion, ensuring that all data is clean and ready for analysis. This makes interpolated data reliable and actionable for decision-makers.
Learn more about how data ingestion in the 5X works!
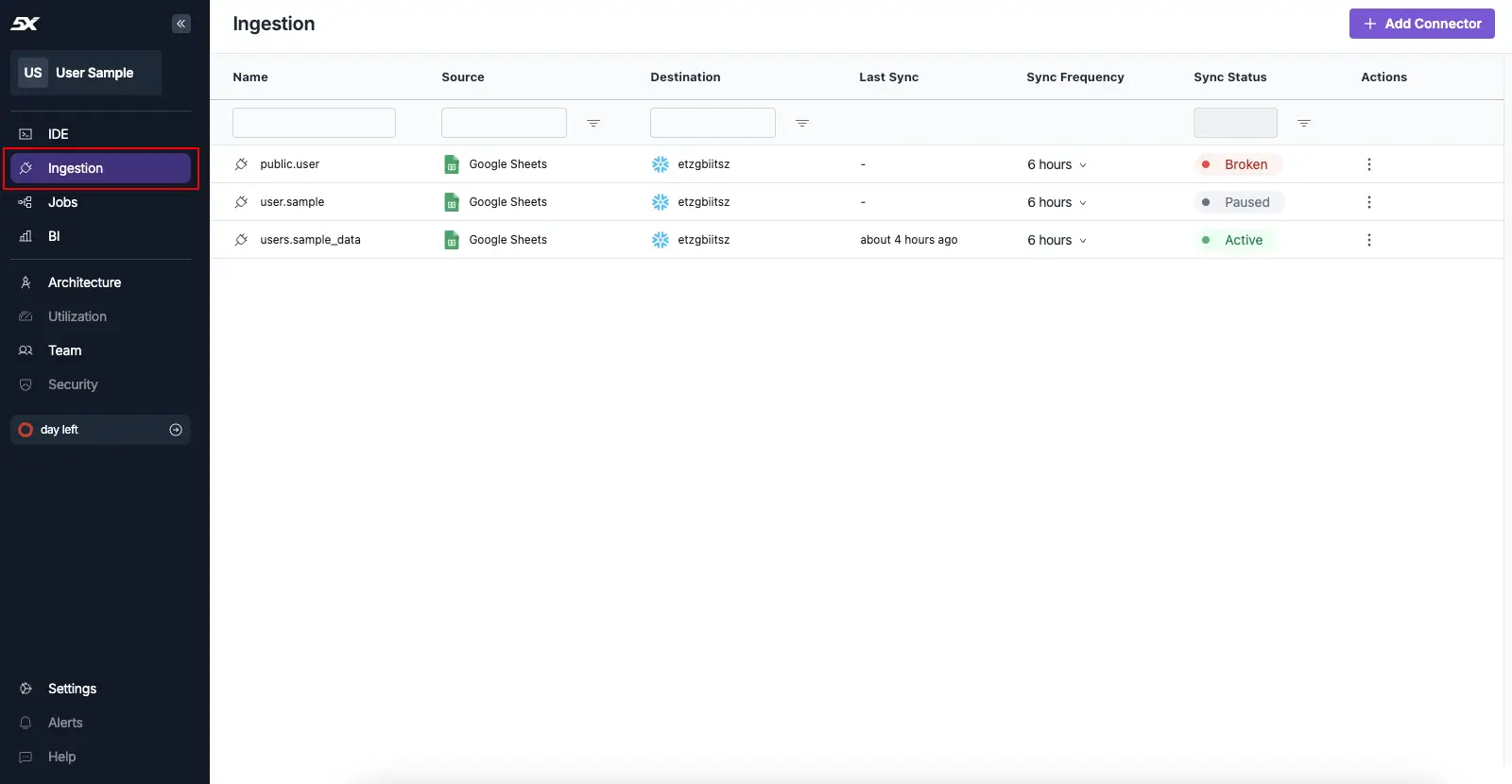
How to interpolate data?
There are multiple methods to perform interpolation, depending on the complexity and type of data you are dealing with. Let’s explore the most common techniques:
1. Linear Interpolation
Linear interpolation assumes a straight-line relationship between two known data points. It’s the simplest method, ideal when the data follows a predictable, linear trend. For example, if you're interpolating sales data from two months, linear interpolation fills in the missing value by averaging the rate of change between the two known points. This technique is frequently used in financial analysis and time series data.
For business users, performing interpolation in Excel is a quick and easy way to apply this method. Excel’s FORECAST.LINEAR function can estimate missing values based on existing data trends.

2. Polynomial interpolation
This method fits a curve through the known data points, providing more flexibility when the relationship between data points isn’t linear. Polynomial interpolation is ideal for data sets with non-linear trends, such as growth curves or seasonal patterns.
However, polynomial interpolation can be prone to overfitting, meaning the curve may match the known data points too closely, making it less reliable for estimating new values. For companies dealing with large, complex datasets, polynomial interpolation may require additional computational power. Leveraging a platform like 5X can help streamline the data consolidation process, making interpolation more efficient.
Modern data warehouses are designed for the cloud. Unlike on-premise solutions, cloud warehouses enable you to scale up and down effortlessly. Learn more about how 5X works with any warehouse, in any way, with any billing.
3. Spline interpolation
Spline interpolation uses multiple polynomial equations to create a smooth curve across data points. Unlike polynomial interpolation, splines avoid overfitting by breaking the data into smaller sections, each handled by its own polynomial equation. This method is especially useful in engineering, scientific research, and analytics. Particularly in situations where smooth transitions between data points are necessary.
4. Interpolation in Excel
Many business users rely on Excel for basic interpolation. Excel provides several built-in functions, like LINEST and TREND, that help with both linear and polynomial interpolation. These tools make it easy to fill in missing data without requiring advanced technical knowledge.
For businesses handling high-volume data, using platforms like 5X ensures that your interpolated data remains clean, accurate, and actionable by simplifying how data is consolidated and prepared for analysis.
Advantages and disadvantages of data interpolation
Advantages:
- Filling gaps in data: Interpolation helps ensure that incomplete datasets become actionable by filling in missing values. Whether you're working with customer data, financial projections, or operational metrics, interpolating data ensures your analysis remains valid.
- Improved decision-making: Complete datasets lead to better decision-making. Interpolated data allows businesses to forecast trends and outcomes more accurately, which is crucial in industries like finance, retail, and logistics.
- Cost and time efficiency: Instead of re-collecting missing data points—which can be costly and time-consuming—interpolation offers an immediate solution for businesses to estimate values based on their existing data.
- Versatile application: Interpolation methods are widely used across different fields, from scientific research to business analytics. Whether you're forecasting future sales or analyzing production metrics, interpolation can be tailored to suit the specific needs of your data
Disadvantages:
- Estimates, not reality: Interpolation estimates unknown values, but the accuracy of those estimates depends on the assumption that the data follows a certain trend. If the actual pattern deviates from the assumptions made, the interpolated values can be inaccurate.
- Overfitting risks: In methods like polynomial interpolation, there’s a risk of overfitting, where the interpolated values are too closely aligned with the known data points, making them less reliable for estimating future or unknown data.
- Computational complexity: For large datasets, certain interpolation techniques, such as spline or polynomial interpolation, can be computationally intensive. This can make them impractical for real-time applications unless you have robust infrastructure like 5X’s warehouse solutions.
Data interpolation plays a crucial role in modern data analytics, providing an effective way to estimate missing data points and make datasets more complete. Whether you’re working with simple time-series data or complex machine learning models, the right interpolation method ensures your data is clean, actionable, and ready for analysis.
While basic tools like Excel can handle linear interpolation for smaller datasets, businesses dealing with large volumes of data or more complex patterns may benefit from using tools like With 5X, you can streamline your data ingestion, consolidate datasets, and improve the accuracy of your interpolated data.
Ready to turn your incomplete datasets into actionable insights? Start with 5X today. The goal is to simplify data ingestion, warehousing, and business intelligence. With 5X, you can ensure that your interpolated data is accurate, reliable, and primed to drive smarter decisions.
What is interpolation in AI?
What is the interpolation method for missing data?
What is the interpolation function in math?
How is interpolation used in data analytics?
What are the key differences between interpolation and extrapolation?
What is the role of data interpolation in machine learning?
Building a data platform doesn’t have to be hectic. Spending over four months and 20% dev time just to set up your data platform is ridiculous. Make 5X your data partner with faster setups, lower upfront costs, and 0% dev time. Let your data engineering team focus on actioning insights, not building infrastructure ;)
Book a free consultationHere are some next steps you can take:
- Want to see it in action? Request a free demo.
- Want more guidance on using Preset via 5X? Explore our Help Docs.
- Ready to consolidate your data pipeline? Chat with us now.
Table of Contents
Get notified when a new article is released
Get an end-to-end use case built in 48 hours
Get an end-to-end use case built in 48 hours
How retail leaders unlock hidden profits and 10% margins
Retailers are sitting on untapped profit opportunities—through pricing, inventory, and procurement. Find out how to uncover these hidden gains in our free webinar.
Save your spot
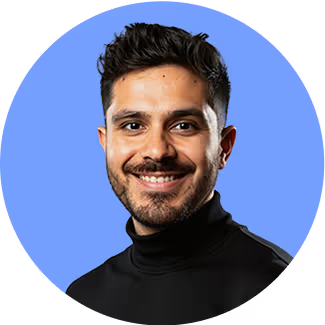