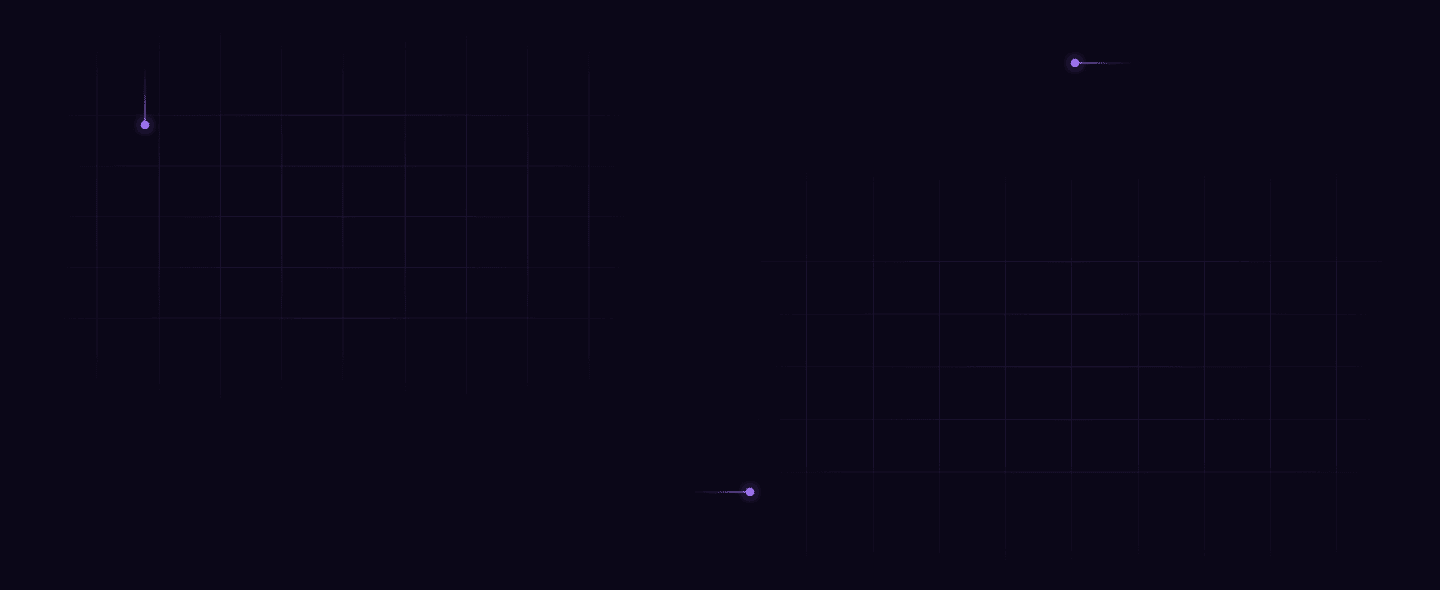
With global data creation projected to grow to 180 zettabytes by 2025, traditional data integration methods are struggling to keep up, creating a white space that AI-driven solutions can fulfill.
AI is revolutionizing the way businesses manage and integrate data. Using AI-driven data integration is not just about automation—it’s about enabling smarter, faster, and more reliable data processing.
This blog delves into the transformative role of AI in data integration, explores its capabilities, and sheds light on how it is reshaping industries with real-world use cases.
What is AI data integration?
According to a recent report by InformationWeek, enterprises with a strong AI strategy are 3 times more likely to report above-average data integration success.
AI data integration refers to the process of leveraging AI and machine learning (ML) technologies to unify, organize, and process data from multiple sources into a single, cohesive view.
Unlike traditional big data integration methods that rely heavily on manual coding and rule-based processes, AI-powered integration uses intelligent algorithms to automate, optimize, and enhance tasks like data extraction, transformation, mapping, and quality checks.
What is the role of AI/ML in data integration?
As organizations strive to become more data-driven, AI/ML is transforming how we approach data integration, making it faster, smarter, and far less tedious.
Traditionally, tasks like mapping, cleaning, and validating data could take hours of manual effort. With AI, these processes are largely automated. ML algorithms can quickly recognize patterns, match schemas, and handle data transformations, freeing teams to focus on more strategic work.
Being data-ready and using AI/ML in data integration helps. Here’s how:
1. Data extraction
AI takes the hassle out of data extraction by automatically analyzing the structure and content of data sources to pull out relevant information. It uses advanced ML)models to recognize patterns and adapt to new data sources, making document processing more efficient and scalable.
Extracting data from unstructured and hierarchical sources—like medical records or financial reports— becomes simpler, especially in industries such as healthcare and finance. This is where AI steps in with Natural Language Processing (NLP). NLP understands the nuances of language, picking out key phrases and terms to extract meaningful insights from unstructured data.
AI also leverages computer vision to extract critical information from images and videos. For example, in healthcare, it can analyze medical imagery like X-rays or MRIs to capture vital diagnostic details, simplifying workflows for medical professionals.
2. Data quality
A study by McKinsey found that organizations leveraging AI in data integration can achieve an average improvement of 20% in data quality.
While traditional big data integration tools have been sufficient to tackle data quality issues, up till now, they can no longer handle the extent of data coming in from a myriad of sources.
One of the biggest wins with AI is improved data quality.
AI catches errors, flags duplicates, and cleans up inconsistencies with impressive accuracy, ensuring that the data you work with is reliable and ready for analysis.
AI driven data integration tools can automatically profile data sources to identify patterns, relationships, and anomalies. AI can also identify and correct errors in data sets and automate the data cleaning process. For example, AI can identify duplicate records or missing data elements and automatically correct them, making data quality management a breeze.
3. Data mapping
Using ML algorithms, AI systems can analyze large data volumes and automatically map data elements from one system to another, saving significant time and resources, particularly when dealing with large and complex data sets.
AI-powered systems can make intelligent AI data mapping suggestions based on historical data and patterns. It utilizes advanced data-matching algorithms that identify matching data elements across different data sources and auto-maps them to the correct location in the target system. These algorithms are useful when dealing with data sources that have different data formats or structures.
What are the benefits of using AI-powered data integration?
Modern data integration (DI) technologies are incorporating AI capabilities into their frameworks to meet the growing demands of enterprises. These advancements are transforming how businesses make data-driven decisions by enhancing speed, usability, and scalability.
Here are the ai data platform benefits
1. Prebuilt mapping and metadata catalog
AI-enabled data integration platforms now offer prebuilt templates and metadata catalogs that simplify the creation of data transformation mappings.
With the 5X data integration platform, non-technical users can leverage drag-and-drop features to perform complex data integration tasks. This empowers domain experts to spend less time on technical operations and more on analyzing data trends and insights relevant to their expertise.
2. Faster computational speed
By leveraging the power of Data Integration in ML (machine learning) , data integration tools can analyze enterprise datasets faster and more efficiently than traditional business intelligence (BI) techniques.
ML algorithms reduce the need for extensive coding while harnessing computational power to deliver actionable insights at unprecedented speeds.
3. Efficient big data processing
Traditional DI tools often struggle to handle the massive scale of big data, particularly when dealing with unstructured or semi-structured formats. AI and ML excel in this domain, parsing through zettabytes of data to uncover hidden business insights. They generate accurate data models and build robust pipelines with minimal human intervention, saving time and resources.
4. Intelligent learning for smarter insights
AI-powered DI platforms enhance the ETL (Extract, Transform, Load) process by automating data transformation mappings. This allows business users to focus on uncovering patterns and trends within large datasets. By applying statistical models to curated data, users can derive precise business insights and make informed decisions without getting bogged down in technical complexities.
AI data integration use cases: How AI data integration is enabling innovation across different industries?
AI data transformation is reshaping industries by enabling seamless data connectivity, improving insights, and driving innovation. Here are industry-specific use cases :
1. Healthcare: Personalized treatment plans
Healthcare systems often juggle data from multiple sources—patient records, diagnostic imaging, lab results, and external research databases. Traditionally, this data has been fragmented, making it challenging to create cohesive patient profiles.
AI-driven data integration eliminates silos by consolidating structured and unstructured data into a unified platform. For example, Natural Language Processing (NLP) extracts valuable insights from doctors’ notes, while image recognition models analyze X-rays, MRIs, and CT scans. This enables physicians to make data-backed decisions and recommend personalized treatment plans.
Example: Cancer treatment centers use AI to integrate genetic data and clinical trial results to recommend targeted therapies for individual patients.
2. Finance: Fraud detection
Banks and financial companies process vast volumes of transactional data daily. Identifying fraudulent activities within this data is critical but challenging without advanced integration systems.
AI-powered data integration combines internal transactional data with external datasets, such as consumer behavior patterns and social media trends. By identifying anomalies in datasets in real-time (such as rapid spikes in credit applications or repeated updates to contact details), AI systems flag potentially fraudulent transactions with unmatched accuracy.
Example: Banks and financial institutions save millions of dollars annually by reducing fraud. Credit card companies like Visa and Mastercard employ AI models that not only detect suspicious activities but also learn from new patterns to improve future detection.
3. E-commerce: Hyper-personalized marketing
Amazon. Walmart. Target. These household giants have taken over the retail and e-commerce landscape, making it extremely difficult for other players to compete in this sector.
Understanding consumer behavior is crucial for success — and AI-based data integration can make all the difference by empowering E-commerce startups and new players to consolidate customer data from websites, mobile apps, social media, and in-store sensors.
These integrated datasets feed AI systems that analyze purchasing habits, preferences, and seasonal trends to create hyper-personalized marketing campaigns. AI also powers recommendation engines, suggesting products that resonate most with individual customers.
Example: Personalized experiences increase customer engagement and boost sales. Companies like Amazon leverage AI-driven data integration to offer highly relevant product recommendations.
4. Manufacturing companies: Predictive maintenance
Manufacturing involves managing extensive machinery and production lines, where downtime can cost millions. AI-driven data integration combines IoT sensor data, machine logs, and maintenance history to monitor equipment health. By analyzing patterns, AI predicts when a machine may fail and schedules repairs proactively, thus reducing downtime and optimizing repair schedules.
Example: Automotive manufacturers use AI to integrate factory data and predict component wear, saving time and resources while enhancing operational efficiency.
5. Logistics companies: Demand forecasting
Logistics and supply chain management rely on accurate demand forecasts to minimize waste and maximize efficiency. AI-based data integration combines historical sales data, real-time inventory levels, weather forecasts, and market trends. Using these integrated datasets, AI models predict demand surges and inventory requirements, ensuring timely stock replacement and distribution.
Example: Walmart uses AI-driven forecasting tools to prepare for seasonal spikes such as holiday shopping periods, reducing instances of overstock or stockouts.
6. Entertainment and media: Content recommendations
Media consumption patterns have shifted dramatically with streaming platforms dominating the industry. AI-based data integration combines user viewing history, ratings, and external data like global trends to suggest relevant content. These systems improve user experience and help platforms understand content popularity to guide future production investments.
Example: Platforms like Netflix and Spotify leverage AI to recommend shows, movies, or songs that resonate with individual users, keeping them engaged for longer.
7. Telecommunications: Network optimization
Telecom providers have to maintain reliable networks while handling growing user demands.
Integrating AI means auto-combining customer usage data, network performance metrics, and real-time alerts to optimize service delivery. These systems can predict and resolve network issues before they impact users, improving service quality and customer satisfaction.
Example: AT&T and Verizon use AI to manage network traffic during high-demand events, ensuring uninterrupted connectivity.
Choosing an AI data integration solution: Factors to consider
Selecting the right AI-powered data integration solution can make or break your business’s ability to harness data effectively. Here are some key factors to consider:
- Scalability and flexibility: Your data needs will grow and evolve. Choose data integration tools that scale effortlessly as your business expands and adapts to handle diverse structured, unstructured, or semi-structured data without requiring complex customizations
- Ease of use: A user-friendly interface is crucial, especially for teams without extensive technical expertise. Features like drag-and-drop workflows, pre-built templates, and intuitive dashboards can empower non-technical users to participate in data integration tasks
- AI capabilities: Ensure the solution offers robust AI and machine learning features, such as automated data mapping, anomaly detection, and predictive analytics. These tools should simplify complex tasks, reduce manual intervention, and speed up insights generation
- Integration with existing systems: The solution must seamlessly connect with your current tools, applications, and data sources—whether on-premises or cloud-based. Look for broad compatibility and robust APIs to avoid disruption
- Security and compliance: With increasing regulations, the solution should prioritize data security and comply with standards like GDPR, HIPAA, or industry-specific requirements. Features like encryption, role-based access control, and audit logs are essential
Cost-effectiveness: Assess the total cost of ownership, including licensing, implementation, and ongoing maintenance. A good solution balances affordability with value
Conclusion
AI is a game-changer in how businesses integrate and utilize data. As organizations face data complexity, volume, and diversity, AI-based data integration tools are stepping up to fill the gaps, offering unprecedented speed, accuracy, and scale.
The advantages are clear: automated data mapping, enhanced data quality, efficient handling of unstructured and big data, and intelligent insights that empower businesses to make data-driven decisions. These tools are not just about streamlining processes but also about enabling innovation.
As AI technology evolves, it will further blur the lines between data integration and actionable insights, making it a strategic asset for businesses across sectors. However, choosing the right AI-powered data integration solution will remain critical.
Leverage 5X to power data integration and unlock business success for your data teams today.
Building a data platform doesn’t have to be hectic. Spending over four months and 20% dev time just to set up your data platform is ridiculous. Make 5X your data partner with faster setups, lower upfront costs, and 0% dev time. Let your data engineering team focus on actioning insights, not building infrastructure ;)
Book a free consultationHere are some next steps you can take:
- Want to see it in action? Request a free demo.
- Want more guidance on using Preset via 5X? Explore our Help Docs.
- Ready to consolidate your data pipeline? Chat with us now.
Table of Contents
Get notified when a new article is released
Get an end-to-end use case built in 48 hours
Get an end-to-end use case built in 48 hours
How retail leaders unlock hidden profits and 10% margins
Retailers are sitting on untapped profit opportunities—through pricing, inventory, and procurement. Find out how to uncover these hidden gains in our free webinar.
Save your spot
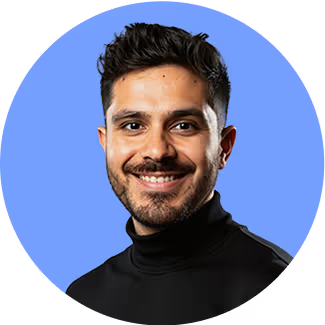