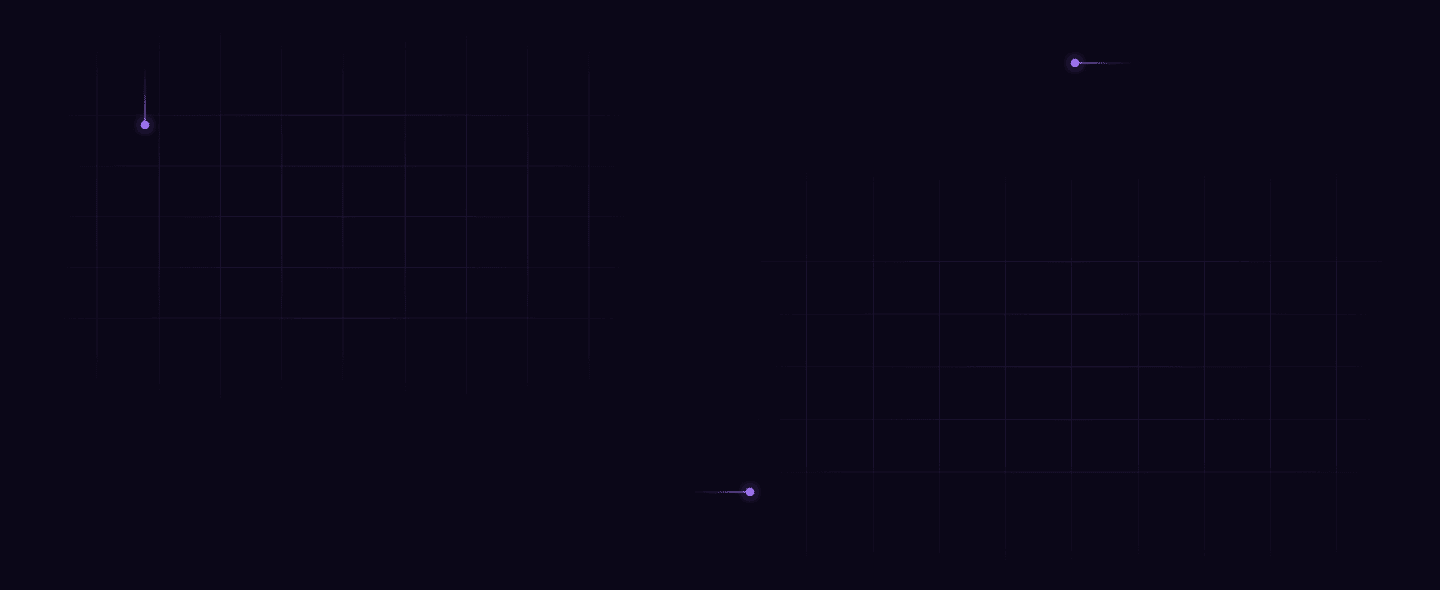
Data teams are under immense pressure. Cost scrutiny is at an all-time high, and the value proposition of data & analytics in driving decision-making can be challenging to quantify in terms of ROI.
Here's the kicker: the data landscape is incredibly fragmented, with over 500 vendors across 30+ categories. This forces companies to pre-select vendors and cobble together a platform. This doesn't help keep costs down or move quickly.
The problem: Traditional "data readiness"—centralizing, cleaning, modeling, and structuring data for business use—was sufficient for BI, reporting, and basic data analytics. However, in the age of AI Data, data readiness takes on a whole new meaning.
Why data readiness matters more than ever: Data readiness is now the cornerstone for powering the entire Gen AI and LLM world. Simply put, you can't have a successful AI strategy without a data strategy. If you don't understand your data, neither will your AI.
So, how do you overcome this challenge and ensure your data platform is ready to support your AI ambitions? By making your data AI-ready!
6-step process to get your data AI-ready
1. Automated Ingestion
Businesses of all sizes struggle with a growing mess of data – from internal apps to customer interactions. This scattered data makes it hard to collect and use effectively. That's why automating data ingestion is crucial. Here's why: manually handling all this data becomes a bottleneck as more sources pop up. Automation ensures everything runs smoothly and your AI projects get the data they need, on time.
2. Engagement Data
For businesses with highly engaging products, there's another data goldmine: usage data! Product analytics or event trackers capture user behavior and store it in a central source. This data is super valuable for AI that personalizes experiences or predicts user behavior.
3. Data Modeling
Using raw data is a bad idea. Its format can change unexpectedly, and it wasn't built for the business in the first place. That's where data modeling comes in. Clean, structure, and model your data so it's reliable and answers your specific business questions.
4. Metrics & Iterations
Once your data is organized, it's time to define what success looks like. Set up a feedback loop with the business stakeholders. Reports for the right stakeholders validate that the metrics defined accurately reflect how the business is looking at them.
5. Semantic Layer
Business stakeholders often lack the technical expertise to navigate complex data reports. A semantic layer can come in handy to standardize metrics definitions. It translates data into easily understandable terms, bridging the gap between technical data and business understanding.
6. Business Process
Invest in a business process to maintain your golden data – a central data team to manage core data sets for consistency across the org and decentralized agile teams that work with departments or product teams to give them the speed to move quickly.
Our next-generation data platform is designed for one thing: making "data readiness" ridiculously easy. Get started in clicks, seamlessly integrate with your existing tools, and scale as your needs grow.
Building a data platform doesn’t have to be hectic. Spending over four months and 20% dev time just to set up your data platform is ridiculous. Make 5X your data partner with faster setups, lower upfront costs, and 0% dev time. Let your data engineering team focus on actioning insights, not building infrastructure ;)
Book a free consultationHere are some next steps you can take:
- Want to see it in action? Request a free demo.
- Want more guidance on using Preset via 5X? Explore our Help Docs.
- Ready to consolidate your data pipeline? Chat with us now.
Table of Contents
Get notified when a new article is released
How retail leaders unlock hidden profits and 10% margins
Retailers are sitting on untapped profit opportunities—through pricing, inventory, and procurement. Find out how to uncover these hidden gains in our free webinar.
Save your spot
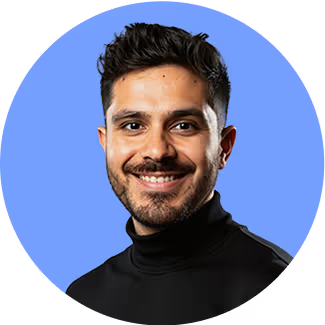