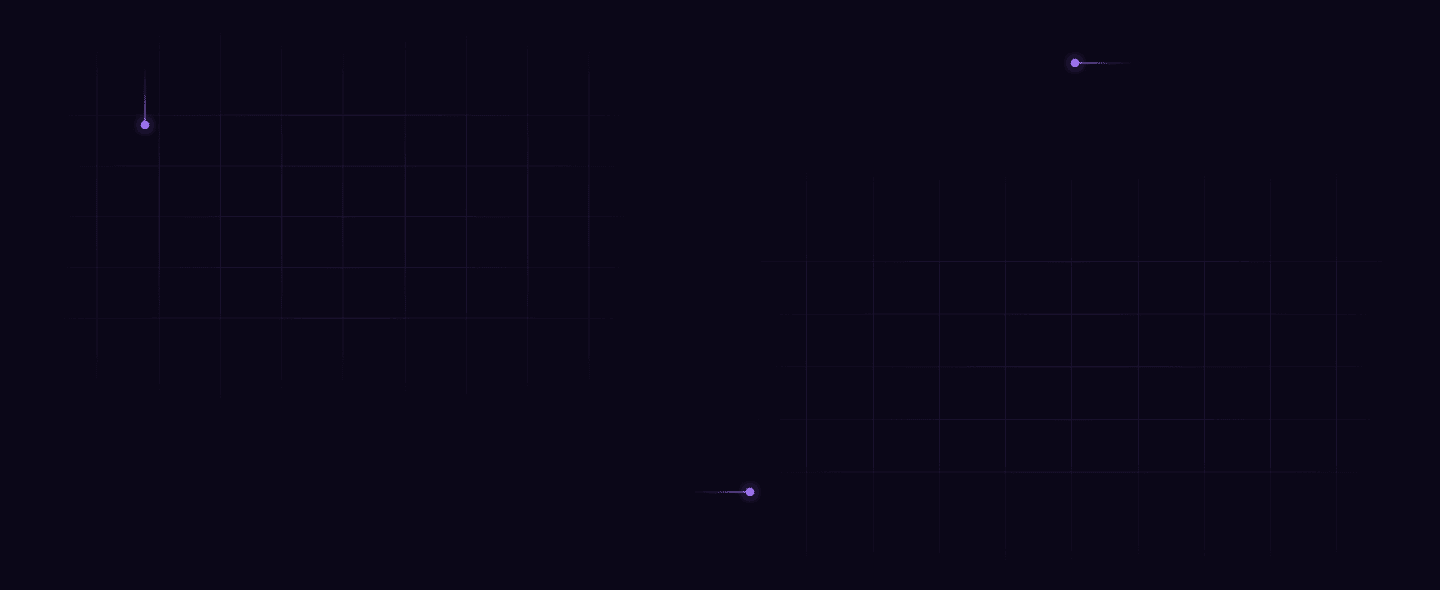
Data Lineage Guide: Definition, Benefits, Use Cases, and Why It Matters For Your Data Teams
.png)
Empower your data teams with impact analysis, ETL pipeline optimization, and accurate feature engineering by implementing data lineage in your business process today.
By tracking each touchpoint and transformation, data lineage enables organizations to understand data’s complete journey from origin to final use. This information is crucial for understanding how data movement transforms your business and where it can make the most impact.
Read this guide to learn about data lineage, its core components, benefits, use cases, and how different teams in your organization can leverage it for business success.
What is data lineage?
Where does data come from?
How is it transformed?
Where is it used?
Knowing the answers to these questions helps a business make informed decisions backed by data.
Data lineage mapping tracks the flow of data from its origin through its entire lifecycle, including various transformations and movements between systems, processes, and users. It gives a detailed trail or "lineage" of data, enabling organizations to understand where data originates, how it moves across the systems, and how it is manipulated and utilized until it reaches its final state.
Data lineage is visualized through diagrams that display the flow and dependencies of data within an organization. This transparency ensures better data governance, compliance, and decision-making.
Data lineage creates a data mapping framework, collects and manages metadata from each step, and stores it in a metadata repository that can be used for lineage analysis. The metadata is updated for each process applied to data in its journey.
What are the core components of data lineage?
Sources, transformations, and dependencies form the core components of data lineage:
- Data origins/sources: Understanding the initial points where your data is created or captured is crucial for ensuring data authenticity and accuracy. These sources can include databases, data lakes, APIs, and even external data providers.
- Data flows and transformations: Data flow is when data moves between systems and processes. Transformations are the changes that happen to data as it moves through different stages of processing. Documenting transformations is vital for understanding how data evolves. Examples include data cleaning, filtering, aggregation, and enrichment.
- Dependencies: The linkage or relationship between different data elements and systems is called a dependency. Identifying dependencies means understanding how changes in one data source or transformation can affect downstream processes and data consumers. It is essential for understanding the impact of changes and for effective data management.
What’s the difference between data lineage and data traceability?
Data lineage and data traceability are often used interchangeably but serve distinct purposes. In simple terms, data lineage gives an overview of how data flows and transforms, while traceability focuses more on verifying and tracking specific data elements throughout their lifecycle.
More specifically, data lineage refers to mapping the flow of data from origin to destination, detailing each transformation step. It helps in understanding the "journey" of the data, including its sources, processing, and outputs. On the other hand, data traceability is about tracking data points back to their source for verification and creating a chain of custody so you know what data came from where and which link would need fixing in case rework is needed.
Another difference is that traceability can be leveraged by any team depending on its needs, while data lineage is only useful for technical users, data product managers, and platform engineers.
Benefits of data lineage: Why is it important?
Implementing data lineage comes with several benefits such as:
- Streamlined troubleshooting: Data lineage provides a clear view of the data journey and identifies the root cause of data quality problems, reducing troubleshooting time
- Regulatory compliance: Data lineage makes it easier to comply with laws and regulations around data collection and usage in the world such as GDPR, CCPA, and HIPAA. With data lineage, companies enjoy a clear view of the data lifecycle and can pinpoint which data should be masked or blocked entirely to maintain confidentiality
- Impact analysis: Analyzing changes in a data field and when they occur is called impact analysis. It helps troubleshoot errors. By making a running record of all data transformations available, data lineage makes it easier to identify the root cause of issues
- Data modeling: Data modeling is about visualizing and cataloging data attributes. Data lineage informs and refines data models by revealing relationships between data elements and providing real-time context into the current flow of data. This information can be used to update previous data models and make them more precise
- Strategic decision-making: Unreliable data can lead to poor decision-making. By creating transparency around its collection, transformations, and storage; data lineage gives you reliable data that you can trust and help you make strategic business decisions
- Boosted collaboration: Data lineage improves collaboration among different teams by providing a shared understanding of data flows and transformations
Is data lineage necessary for every data team?
For smaller teams dealing with limited datasets, data lineage might not be a critical priority. However, as the data ecosystem grows in complexity and volume, data lineage becomes essential.
For large organizations with multiple data sources and complex data pipelines, data lineage is crucial as it ensures data reliability, traceability, and compliance. It helps data teams understand data dependencies, detect anomalies, and maintain data quality across various systems.
So while data lineage is not mandatory for every data team, many teams can benefit from its capabilities. Here’s a detailed overview:
1. Data governance teams
- Ensure compliance: Track data movement and transformations to comply with regulations like GDPR, HIPAA, and SOX
- Data quality management: Monitor data flow to identify quality issues and ensure consistent, accurate data across the organization
- Establish data policies: Create and enforce data management policies using a clear view of data origins and transformations
2. Data engineering teams
- Optimize ETL pipelines: Understand how data flows through ETL (Extract, Transform, Load) processes to enhance pipeline efficiency and minimize errors
- Debug data issues: Trace the origin of data discrepancies quicker to enable fast troubleshooting
- Impact analysis: Assess the downstream impact of changes in data sources or transformations on analytics and reporting
3. Business intelligence (BI) and analytics teams
- Improve reporting accuracy: Verify the source and transformations of data used in reports and dashboards to enhance reliability
- Increase trust in insights: Provide transparency into data origins, increasing stakeholders' confidence in the insights derived from data lineage tools for business intelligence
- Efficient root cause analysis: Quickly identify and resolve data issues affecting dashboards or reports by tracing data lineage
4. Data science teams
- Enhance model accuracy: Ensure the quality of input data for machine learning models by understanding its lineage and transformation history
- Feature engineering: Track the transformations and derivations of features used in models to replicate and verify results
- Experimentation and analysis: Understand how data flows and changes over time, helping in hypothesis testing and model improvement
5. Compliance and risk management teams
- Audit trail creation: Establish a clear audit trail of data, aiding in regulatory compliance and internal audits.
- Risk mitigation: Identify potential sources of risk by understanding data flows and how data is transformed across systems
- Regulatory reporting: Ensure accurate data is used in regulatory reporting by tracing its origin and transformation path
6. IT and data operations teams
- System monitoring and maintenance: Monitor data flows across systems to ensure smooth operations and identify bottlenecks.
- Incident management: Quickly locate the source of issues in data pipelines to reduce downtime and improve system reliability
- Data security: Track sensitive data across systems to ensure it is handled securely and in compliance with data privacy regulations.
7. Product management teams
- Informed decision-making: Leverage insights from data lineage to understand how product data is collected and transformed for accurate analysis
- Feature development: Assess the impact of new product features on data pipelines and reporting metrics
- Data-driven roadmap: Use lineage insights to prioritize product enhancements based on reliable data insights
8. Data stewardship teams
- Maintain data consistency: Ensure that data definitions and formats are consistent across the organization by monitoring lineage
- Data cataloging: Enhance data cataloging by including lineage information, providing users with a complete view of data sources and transformations
- Data transparency: Increase transparency and trust in data by providing detailed lineage information to stakeholders
What are the use cases of data lineage?
By investing in data readiness tools and strategies, businesses can optimize their data management practices, gain deeper insights, and maintain a competitive edge in their respective markets.
Data lineage is beneficial across various industries and use cases such as:
1. Financial services: Ensuring compliance and accurate reporting
In the financial services industry, regulatory compliance is a critical concern.
Laws such as the Sarbanes-Oxley Act (SOX), Dodd-Frank Act, and Basel III require organizations to maintain detailed audit trails and ensure data integrity.
How data lineage helps:
- Audit Trail: Provides a clear path of how financial data is collected, processed, and reported, making it easier for companies to meet audit requirements.
- Risk Management: Helps in identifying discrepancies in data, reducing financial risks.
- Data Accuracy: Ensures that financial reports are generated using accurate and consistent data, which is crucial for compliance.
Examples of how financial services companies can leverage data lineage:
- JP Morgan Chase can use data lineage to comply with regulatory requirements, trace data sources, and maintain accurate financial reporting
- Goldman Sachs can implement data lineage for risk management and regulatory compliance, ensuring the accuracy of their trading and investment data
- HSBC can utilize data lineage tools to trace data flow and transformations to fulfill complex audit and compliance requirements
2. Healthcare: Ensuring patient data accuracy and regulatory compliance
The healthcare industry deals with sensitive patient data that should be handled with strict privacy and accuracy due to regulations like HIPAA (Health Insurance Portability and Accountability Act).
How data lineage helps:
- Data privacy compliance: Tracks patient data across systems to ensure compliance with regulations such as HIPAA and GDPR
- Improved data quality: Identify data errors or inconsistencies that can affect patient care
- Interoperability: Provides a clear view of how patient data moves across different systems (EMRs, labs, billing systems), enhancing data sharing and integration
Examples of how healthcare companies can leverage data lineage:
- Mayo Clinic can use data lineage to track patient records across various healthcare systems, ensuring data consistency and compliance with regulations
- Cerner Corporation can implement data lineage in its electronic health records (EHR) systems to maintain accurate patient data and streamline healthcare operations
- Pfizer can utilize data lineage to ensure compliance with clinical trial data regulations and maintain the integrity of research data
3. Retail: Enhancing customer insights and marketing personalization
Retailers collect vast amounts of data from multiple sources, such as point-of-sale (POS) systems, e-commerce websites, and customer loyalty programs. Understanding customer behavior and preferences is key to delivering personalized experiences.
How data lineage helps:
- Customer data flow: Provides visibility into how customer data is collected, processed, and used across various systems, helping retailers gain accurate insights into customer behavior
- Personalized marketing: Ensures that customer segmentation or purchase history data is accurate and reliable, enabling targeted campaigns
- Data integration: Integrates data from various sources, such as online and offline channels, to create a unified customer view
Examples of how retail companies can leverage data lineage:
- Walmart can use data lineage to trace and analyze customer data from multiple channels to improve inventory management
- Amazon can implement data lineage to ensure accurate customer insights and enhance its recommendation engine to drive personalized experiences for shoppers
- Nike can utilize data lineage to integrate and analyze customer data across different touchpoints to enable targeted marketing and better customer engagement
4. Manufacturing: Enhancing supply chain visibility
In the manufacturing industry, understanding the flow of data across the supply chain is critical for optimizing operations and ensuring product quality.
How data lineage helps:
- Supply chain transparency: Tracks data from suppliers, production processes, and distribution to provide end-to-end visibility
- Quality control: Identifies data inconsistencies or errors that might affect product quality, allowing for timely corrective actions
- Regulatory compliance: Ensures compliance with industry standards by maintaining accurate records of data flow and product specifications
Examples of how manufacturing companies can leverage data lineage:
- Toyota can use data lineage to trace where parts and materials are sourced from across the supply chain, improving quality control and compliance with industry regulations
- Unilever can implement data lineage to optimize its supply chain processes and ensure accurate tracking of raw materials and production data
- Siemens can leverage data lineage for real-time monitoring of manufacturing processes and enhance transparency and operational efficiency
5. Pharmaceuticals: Ensuring data integrity in clinical trials
The pharmaceutical industry relies heavily on accurate data during clinical trials and drug development. Establishing data lineage helps maintain the integrity of research data and ensures compliance with regulations like FDA guidelines.
How data lineage helps:
- Data integrity: Tracks the flow of data collected during clinical trials to ensure its accuracy and reliability
- Regulatory compliance: Provides an audit trail of research data, fulfilling requirements set by regulatory bodies such as the FDA
- Risk mitigation: Identifies potential data discrepancies early-on to reduce the risk of errors in drug development processes
Examples of how pharmaceutical companies can leverage data lineage:
- Johnson & Johnson can utilize data lineage to ensure the integrity of data collected during clinical trials, improving compliance and accuracy
- Roche can implement data lineage to trace data from multiple sources in its research processes, enhancing data quality and compliance
- Novartis can use data lineage to monitor and verify the flow of clinical data, thus ensuring data reliability for regulatory submissions
How can you perform data lineage strategically?
Different approaches to performing strategic data lineage include:
1. Lineage by data tagging
This approach is about tagging your data as it moves through different systems. Think of it as adding little sticky notes to your data that tell you where it came from, what changes it's gone through, and how it’s been used. As data flows through different stages, you can keep adding metadata tags that capture more details about its journey. The key here is to have a standardized set of tags so everyone knows what each tag means, making it easier to track data changes effectively.
2. Self-contained lineage
Self-contained lineage is like keeping a diary for a specific data set within a single system. It tracks everything that happens to the data inside one platform but doesn’t follow it when it moves to another system. So, if your data stays put in one place, this method works great for capturing its full history without worrying about how it interacts with other applications or systems.
3. Lineage by parsing
Lineage by parsing is about analyzing and breaking down your data sources—like tables or log files—to get a clear picture of how the data has transformed. Imagine you have a complex spreadsheet; parsing would be like simplifying it into a format that’s easy to understand and documenting those changes for future reference. It’s like translating data from its complicated form into a more digestible version while keeping a record of the process.
4. Pattern-based lineage
Instead of tracking every single data movement individually, pattern-based lineage looks at common trends or repeated data changes. It identifies recurring patterns and uses them to map out the history of multiple data sets. Rather than focusing on each piece of data, it monitors similar changes across different data assets, making it easier to understand data flows based on recurring behaviors.
Data lineage best practices to get more value from data lineage
Some data lineage best practices you should follow are:
1. Prioritize quality over quantity
When setting up data lineage, it’s better to track fewer and higher-quality data elements that matter. Instead of capturing every data, focus on those that impact business decisions. This gives you a clearer picture and helps you spot and fix any inconsistencies that could disrupt your operations.
2. Highlight what’s important with field-level data lineage
Field-level data lineage zooms in on specific data points—like customer names or transaction amounts—and shows how they move and change across different systems. By honing in on these critical fields, you can quickly identify issues and understand how specific data is used. This approach leads to better data quality and helps teams fix problems faster.
3. Organize data lineage for easy understanding
When data lineage is well-organized, it is easier to understand information, spot insights, and make informed decisions. Using clear diagrams, data lineage visualation maps, and standardized formats can simplify complex data flows, making them accessible to data teams, analysts, and non-tech business users.
4. Add the right context to your data lineage
Data lineage isn’t just about showing where data came from and where it’s going; it’s also about explaining why and how it changes along the way. Including details like metadata, business rules, and transformation logic adds context, helping everyone understand the full story behind the data. Such transparency is crucial for compliance, governance, and smart business decisions.
5. Scale data lineage to keep up with business growth
As your data landscape grows, your data lineage needs to grow with it. Implementing automated tools and scalable infrastructure can help manage increasing data volumes and complexity. Investing in systems like 5X helps you expand your business and ensures that your data lineage continues to provide valuable insights as your needs evolve.
Conclusion
Data governance to BI, analytics, data governance, and improving decision-making are some of the few advantages of implementing data lineage within an organization. By strategically adopting different data lineage approaches such as data tagging or pattern-based lineage, businesses can gain deeper insights and ensure better quality control.
In summary, data lineage is a critical component of modern data management, enabling companies to harness the full potential of their data assets and maintain a competitive edge.
By offering end-to-end data visibility, automated data lineage mapping, and enhanced governance, 5X leverages data lineage principles and helps you make a real business difference.
What’s the difference between data lineage and data flow?
Why do you need data lineage?
What happens if you don’t establish the lineage of the data you’re using?
What’s the difference between data lineage and data audit?
How would you explain data lineage?
What are data lineage diagrams?
Building a data platform doesn’t have to be hectic. Spending over four months and 20% dev time just to set up your data platform is ridiculous. Make 5X your data partner with faster setups, lower upfront costs, and 0% dev time. Let your data engineering team focus on actioning insights, not building infrastructure ;)
Book a free consultationHere are some next steps you can take:
- Want to see it in action? Request a free demo.
- Want more guidance on using Preset via 5X? Explore our Help Docs.
- Ready to consolidate your data pipeline? Chat with us now.
Table of Contents
Get notified when a new article is released
Get an end-to-end use case built in 48 hours
Get an end-to-end use case built in 48 hours
Wait!
Don't you want to learn how to quickly spot high-yield opportunities?
Discover MoonPay’s method to identify and prioritize the best ideas. Get their framework in our free webinar.
Save your spot

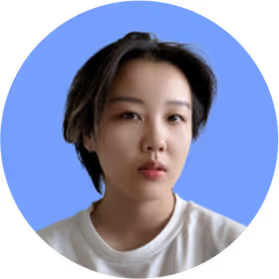