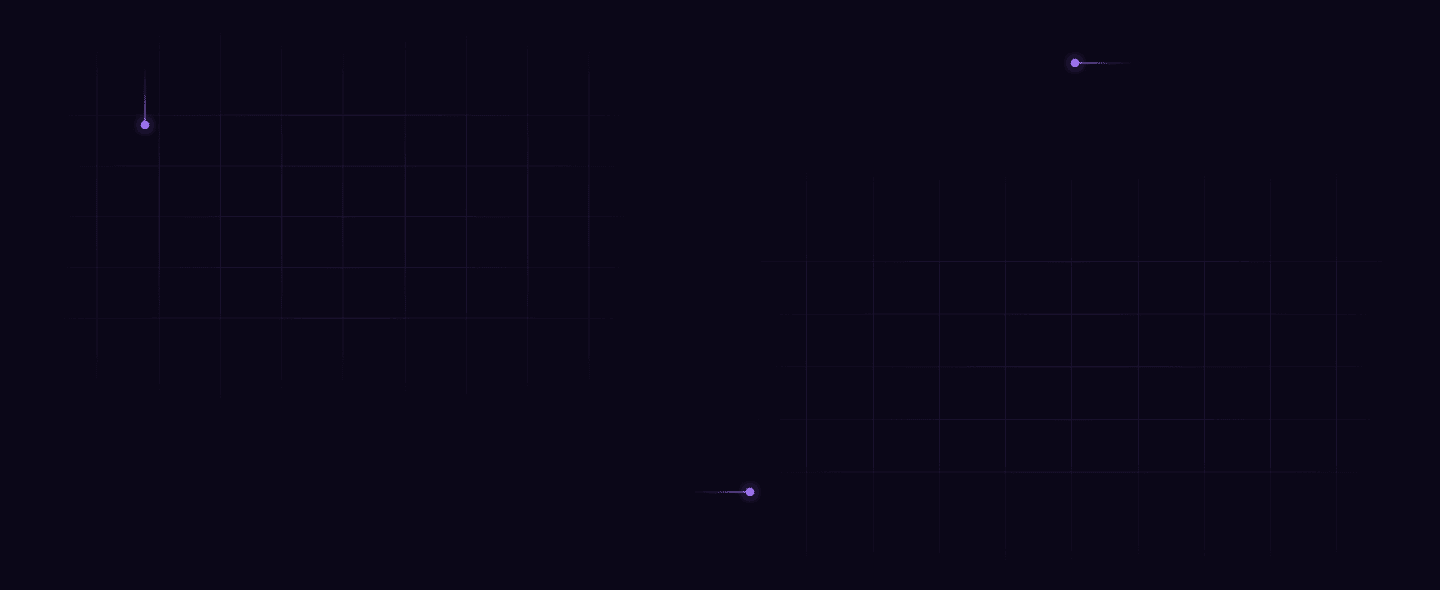
Modern manufacturing is driven by data. With AI-powered analytics, manufacturers can improve product quality, reduce downtime, and predict demand with incredible accuracy.
Data science is a game-changer in manufacturing. When harnessed correctly, data science can predict demand, enhance quality control, minimize downtime, and refine supply chain operations.
In this blog, we’ll explore the real-world applications, benefits, challenges, and opportunities of implementing data science in the manufacturing process.
What is data science in manufacturing?
The journey of manufacturing a product begins with an idea. Then comes design, material selection, machinery, skilled workers, packaging, and supply chain logistics.
Every step in the manufacturing process matters as it impacts cost, efficiency, and output. With the intervention of data science, you can optimize each step.
Applying data science in manufacturing empowers manufacturers to analyze large volumes of data from sensors, machines, and production lines to gain valuable insights. This results in optimized production, improved product quality, reduced waste, and enhanced efficiency.
Role of data science in manufacturing
On the business intelligence side, data science helps manufacturers make smarter decisions. It predicts demand, optimizes inventory, sets optimal pricing, and identifies trends before they happen.
On the factory floor, data science drives efficiency. Done right, it can streamline operations, reduce waste, and transform the way manufacturers work.
Types of data generated in the manufacturing process
Manufacturing generates vast amounts of data with each playing a crucial role in optimizing operations. Here’s how data science transforms data sources into actionable insights:
- Sensor data: IoT sensors on machines track temperature, pressure, and vibration. Using this data, manufacturers can predict equipment failures and schedule maintenance before a breakdown
- Machine data: Machines continuously generate data on usage, speed, and energy consumption. Analyzing this data helps pinpoint inefficiencies, identify bottlenecks, monitor equipment performance, and optimize overall production efficiency
- Production data: Using data science automates the process of tracking machine performance, production output, cycle times, defect rates, material usage, and labor efficiency. By analyzing this data, companies can adjust production schedules, capture output rates, downtime, and productivity trends, allowing manufacturers to streamline operations
- Quality data: Comes from inspections and testing during production. Data analytics helps detect patterns in defects, leading to quicker identification of issues
- Inventory data: Monitors stock levels and materials usage. Using data science, manufacturers can develop predictive models to suggest optimal times for restocking, reducing carrying costs
- Maintenance data: This includes logs of past repairs and servicing schedules. AI-driven predictive maintenance helps schedule upkeep proactively, preventing costly unplanned downtimes
- Process data: Captures information about manufacturing workflows, such as the time taken for each production step. This data can reveal inefficiencies and suggest improvements for faster, smoother operations
- Supply chain data: Supply chain data gives insights into supplier reliability, logistics, and the flow of materials from origin to factory. By applying data science to this data, manufacturers can optimize routes, reduce transportation costs, and increase material supply reliability
- Customer feedback: Reviews, complaints, and product return data provide insights into market expectations. Analyzing this data helps improve future product designs and forecast demand more accurately
Ten applications of data science in manufacturing
Here are ten powerful ways data science is transforming manufacturing businesses stay competitive:
1. Quality control
Manufacturers use AI-powered computer vision to improve quality control. Detecting small defects like cracks, scratches, or shape variations is difficult with manual inspection. Using AI models that are trained to recognize these flaws make the process faster and more accurate.
Computer vision also ensures precise measurements, reducing errors and saving time. By automating inspections, manufacturers cut costs, improve efficiency, and deliver higher-quality products.
2. Demand forecasting
Overproduction wastes resources. Underproduction leads to missed sales.
Accurate demand forecasting solves both problems.
Using advanced analytics, data scientists clean and analyze historical sales, market trends, and financial data. Machine learning algorithms then analyze these patterns to predict future demand with high accuracy. These AI-driven models continuously refine their predictions and adapt to changing market conditions in real-time.
With precise demand forecasting, manufacturers can optimize production schedules, prevent supply chain disruptions, and maintain ideal inventory levels. This results in lower storage costs and improved cash flow.
3. Inventory management
Manufacturing companies cannot make accurate financial estimates without efficiently managing their inventory. Data science is a game-changer as it enables precise forecasting using statistical tools. This ensures that inventory does not exceed what's currently required or falls short of what’s needed.
By accurately predicting demand using data science, manufacturers optimize inventory, reduce waste, and save costs. This approach keeps operations efficient and cost-effective.
4. Product design and development
Products are often developed based on customer demand in the market and improved whenever feedback is received. This process is mainly based on designer experience, trial, and error, and a prototype model approach that requires a huge amount of time and some risk of failure.
Data science has revolutionized product designing. Using new designing software like CAD and simulation software like MATLAB makes product design and development quicker, more accurate and reliable.
5. Supply chain optimization
A strong supply chain keeps production smooth and customers happy. But managing inventory, vendors, and deliveries efficiently is a challenge. That’s where data science steps in.
By analyzing supply schedules, stock levels, and vendor performance, manufacturers can prevent shortages and avoid excess inventory. Advanced tracking tools like RFID and barcode scanning provide real-time updates, allowing companies to monitor shipments and warehouses remotely.
With data-driven insights, manufacturers can optimize logistics, reduce delays, and cut costs. A smarter supply chain means better efficiency, faster deliveries, and a competitive edge in the market.
6. Price optimization
Setting the right price is key to selling a product. It should be competitive, fair, and aligned with customer expectations. The challenge is that factors like seasonal shifts, supply shortages, and transportation costs cause price fluctuations, which makes it difficult to decide the right price.
Data science analyzes past and current trends to predict future prices with high accuracy. This empowers businesses to anticipate market changes and adjust pricing strategies.
7. Predictive maintenance
Traditional maintenance strategies rely on either reactive repairs, which involves fixing equipment after a breakdown, or scheduled maintenance, where machines are serviced at fixed intervals regardless of their actual condition. Both approaches lead to inefficiencies.
While reactive maintenance causes unexpected downtime and costly repairs, scheduled maintenance may result in unnecessary servicing, increasing operational expenses.
Predictive maintenance, powered by data science, transforms this process by using real-time monitoring and AI-driven analytics. Sensors embedded in machinery track key parameters such as temperature, pressure, vibration, and energy consumption. This data is continuously analyzed using machine learning models, which identify subtle patterns and anomalies that signal potential failures before they occur.
Moreover, AI models analyze this data to detect early signs of failure. This allows manufacturers to fix issues before they cause downtime. This results in lower costs, higher uptime, and extended equipment life.
8. Market price prediction
Pricing is complex. It shifts with raw material costs, market demand, competitor pricing, seasonality, and global supply chain disruptions. Setting the right price requires a deep understanding of these variables to maximize profitability while remaining competitive.
Data science helps manufacturers set the right price at the right time. It transforms pricing strategies by leveraging advanced analytics and machine learning models. Historical pricing data, consumer behavior, and economic trends are fed into predictive models that use decision trees, regression analysis, and deep learning algorithms. These models identify hidden patterns and correlations that may not be apparent through traditional analysis.
9. Fault prediction and preventive maintenance
Machines generate massive amounts of data through sensors tracking temperature, speed, humidity, and vibration. When these values go beyond safe limits, product quality suffers, leading to returns, rework, or scrapped goods.
All of this costs money.
Unmonitored machines are also at risk of sudden breakdowns, causing downtime and lost production.
Predictive maintenance helps as computer vision improves maintenance by spotting defects in machine parts that need replacement. On the other hand, quality control tools like statistical sampling ensure that the best products reach customers. Using fault prediction and preventive maintenance, manufacturers can reduce downtime, lower costs, and improve customer satisfaction, all thanks to data science.
10. Warranty claim analysis
Warranties assure customers of a defect-free product for a specified period, but managing claims can be a significant financial burden for manufacturers. Fraudulent claims, excessive payouts, and inefficient tracking systems can lead to revenue losses and operational inefficiencies.
With a data-driven approach, manufacturers can enhance customer satisfaction, optimize resource allocation, and make warranty management more cost-effective and transparent. Using predictive analytics, manufacturers can identify recurring defects and improve product design, ultimately reducing future claims.
Benefits of implementing data science in manufacturing:
Implementing data science in manufacturing offers numerous benefits, including:
- Improved quality control: AI-powered computer vision and predictive analytics detect defects early, reducing waste and ensuring consistent product quality
- Optimized inventory management: Data-driven forecasting prevents overstocking and stockouts, reducing storage costs and improving cash flow
- Increased efficiency: Automation and AI optimize production schedules, streamline operations, and minimize downtime
- Predictive maintenance: Sensors and machine learning models identify potential equipment failures before they happen, reducing unplanned downtime and repair costs
- Enhanced demand forecasting: Analyzing market trends and customer data helps manufacturers produce the right amount at the right time, reducing waste and lost sales
- Supply chain optimization: Real-time data tracking ensures smooth logistics, minimizes delays, and improves vendor performance
- Cost reduction: Data science-driven insights help cut production inefficiencies, reduce material waste, and lower operational expenses
- Competitive advantage: Smarter decision-making, faster innovation, and better customer satisfaction position manufacturers ahead of competitors
Challenges and opportunities of data science in manufacturing
Data science is revolutionizing manufacturing. It’s enhancing how products are made, optimized, and delivered, often at more competitive prices. However, adopting data science and implementing it in manufacturing comes with challenges that must be addressed to unlock its full potential.
Here’s a quick overview of the challenges and opportunities of implementing data science in the manufacturing industry:
1. Cultural resistance
Change is often met with resistance from traditional manufacturing companies that are too used to manual processes. Paired with software and infrastructure upgrades, these barriers often deter businesses including smaller firms from adopting a data-centric model.
Aligning data science objectives with the organization's broader mission helps mitigate these hurdles. Stakeholder buy-in and a well-defined strategy bridges the gap and makes all the difference.
2. Data silos and integration challenges
Leveraging data science to fine-tune manufacturing includes working collaboratively. Information scattering across teams results in data silos which often complicates the process.
Implementing a unified data architecture that fosters information-sharing across the organization can help. By standardizing data formats and adopting robust data governance frameworks, manufacturers can work collaboratively and implement actionable insights.
3. Talent gap and the crucial role of data scientists
Data science demands specific skills for effective implementation. A key challenge is the scarcity of highly skilled data scientists, a sought-after role across various industries.
Investment in workforce development by upskilling existing staff and hiring data science experts can empower the organization and make it adaptable to rapid technological changes.
Conclusion: Success in manufacturing is driven by data science
As manufacturing continues to evolve, those who embrace data science will unlock higher efficiency, better product quality, and greater profitability.
In the manufacturing landscape, efficiency and precision are the keys to success, and data science is the driving force behind both. However, leveraging data science effectively requires going beyond information collection and going the extra mile to transform insights into action.
This is where leveraging 5X’s data platform comes in. By providing real-time accessibility, seamless integration, and scalable analytics, 5X enables manufacturers to harness the full power of their data without complex engineering efforts. Talk to our experts to learn how you can embrace data science with our full-stack data solutions platform.
Can data science improve product quality and lower defect rates?
Can data science boost sustainability in manufacturing operations?
How can smaller manufacturers leverage data science?
What does a data analyst do in a manufacturing company?
Building a data platform doesn’t have to be hectic. Spending over four months and 20% dev time just to set up your data platform is ridiculous. Make 5X your data partner with faster setups, lower upfront costs, and 0% dev time. Let your data engineering team focus on actioning insights, not building infrastructure ;)
Book a free consultationHere are some next steps you can take:
- Want to see it in action? Request a free demo.
- Want more guidance on using Preset via 5X? Explore our Help Docs.
- Ready to consolidate your data pipeline? Chat with us now.
Table of Contents
Get notified when a new article is released
Get an end-to-end use case built in 48 hours
Get an end-to-end use case built in 48 hours
How retail leaders unlock hidden profits and 10% margins
Retailers are sitting on untapped profit opportunities—through pricing, inventory, and procurement. Find out how to uncover these hidden gains in our free webinar.
Save your spot
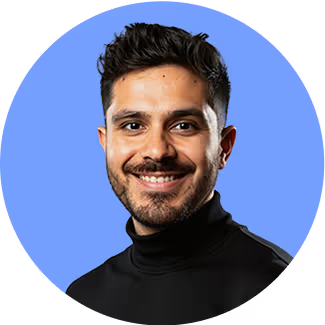