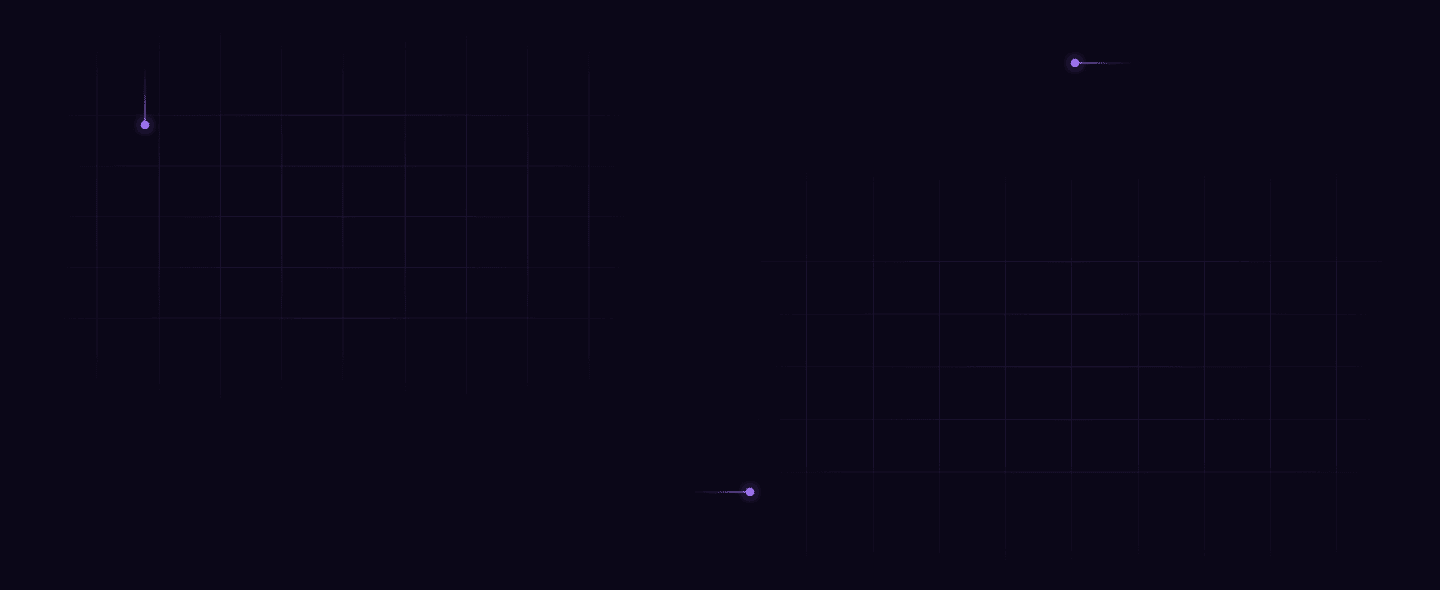
7 Trusted Snowflake Alternatives for 2024
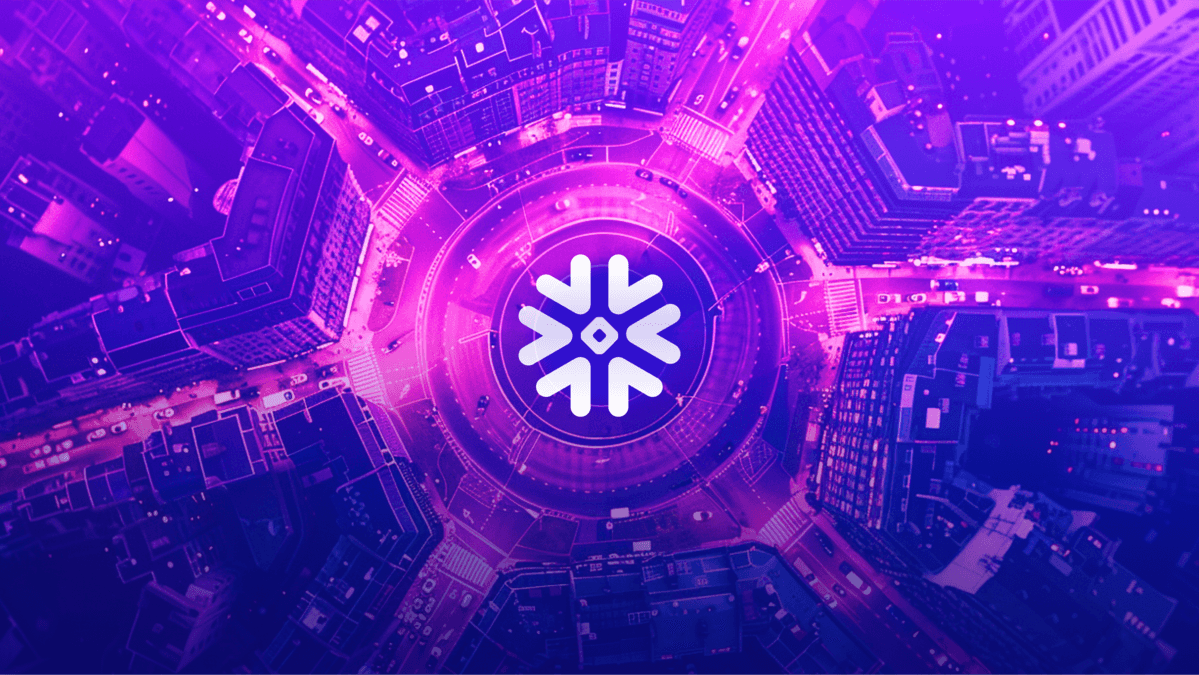
Snowflake is often hailed as the ultimate data warehousing solution, but what if it’s not the best fit for everyone?
Sure, it’s great at handling massive data volumes and has some sleek features. But it’s not a catch-all solution.
For starters, Snowflake’s pricing can get out of hand, especially with heavy workloads and extensive data usage.
Plus, it can be cumbersome for troubleshooting complex errors and requires third-party tools to build a complete data analytics stack.
It also struggles in data ingestion, modeling, and orchestration. And let’s face it, having a data warehouse isn’t everything—being truly data-ready is key.
These factors can drive users to look for other options.
Are there other tools that do a better job? There are indeed. While most alternatives focus on warehousing and lakehouses, there's one that really tackles data readiness.
In this article, we’ll explore 7 Snowflake alternatives and explain why true data readiness is more than just storage. Stick around to see where Snowflake really falls short at.
Top 7 Snowflake alternatives for every use case
Warehousing platforms
Amazon Redshift
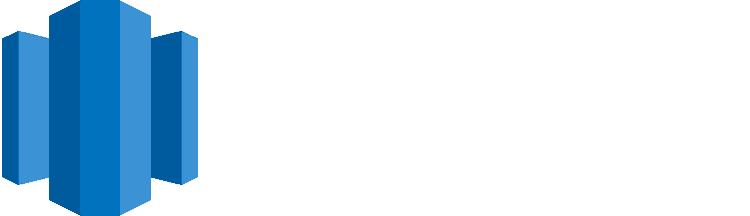
Amazon Redshift is a fully managed data warehouse service in the cloud, designed for large-scale data analysis and reporting. It's a part of the larger AWS cloud-computing platform.
Redshift is a strong alternative to Snowflake for businesses already on AWS. It offers similar data warehousing power and scales well for big data. Plus, Redshift can be more cost-effective, particularly for basic tasks.
Key features
- Columnar storage: Optimizes storage and query performance.
- Massively parallel processing (MPP): Distributes query loads across multiple nodes, similar to Snowflake’s multi-cluster shared data architecture.
- Redshift Spectrum: Queries data directly in S3 without loading it into Redshift, comparable to Snowflake's ability to query external stages.
- Concurrency scaling: Automatically adds resources to handle concurrent queries, akin to Snowflake's automatic scaling of virtual warehouses.
- Advanced security: VPC, encryption, and IAM integration, similar to Snowflake's end-to-end encryption and advanced security features.
- Materialized views: Speeds up complex queries by storing precomputed results, like Snowflake's materialized views.
- Data sharing: Securely shares live data with Redshift clusters and AWS services, whereas Snowflake offers secure data sharing across accounts.
Advantages
- Speed beast: Redshift is incredibly fast. Running queries on massive datasets feels smooth and the results come back quickly.
- Scaling up with ease: Adding more processing power is straightforward, which is great as your data grows.
- Surprisingly manageable: Even without a dedicated database admin, managing Redshift isn't tough. The AWS documentation is a big help here.
- Plays well with others: Being part of the AWS ecosystem, Redshift integrates seamlessly with other AWS services, making data workflows a breeze for AWS users.
- Cost-conscious option (with Serverless): Redshift Serverless seems like a good way to keep costs under control by only paying for what you use.
Disadvantages
- Cluster care can be a chore: Managing Redshift clusters and resources can get complex at times.
- Cost can bite: Especially for startups, Redshift can be expensive. You need to keep a close eye on the budget.
- UI needs work: The user interface could be more user-friendly. Those autosuggestions in the query editor are more annoying than helpful.
- Missing a few pieces: Redshift seems to lack some built-in functions compared to other databases, and adding schema names to queries feels clunky.
- Limited cross-cloud support: Primarily designed for AWS, with limited multi-cloud capabilities, whereas Snowflake supports AWS, Azure, and GCP.
Should you consider Redshift?
If cost is a major concern and you're already heavily invested in AWS, Redshift can be a viable option. However, for new users or existing Snowflake users who prioritize cloud neutrality, advanced features, scalability, and ease of use, Snowflake might still be the better choice.
Google BigQuery (GBQ)
.png)
Google BigQuery is a serverless, highly scalable, and cost-effective multi-cloud data warehouse designed for business agility.
For cost-conscious users or those comfortable with SQL, Google BigQuery is a strong alternative to Snowflake. Its user-friendly interface and tight Google Cloud integration make it a good choice, especially if you already use Google services.
Key features
- Serverless architecture: No infrastructure to manage, unlike Snowflake which uses virtual warehouses.
- Real-time analytics: Supports real-time data streaming, similar to Snowflake’s Snowpipe for continuous data ingestion.
- BigQuery ML: Built-in machine learning capabilities, whereas Snowflake integrates with external ML tools like DataRobot and H2O.ai.
- Integration with Google Cloud: Seamless integration with Google Cloud services, akin to Snowflake's integrations with various third-party tools and platforms.
- Data partitioning: Automatic partitioning of large datasets for optimized queries, comparable to Snowflake’s micro-partitioning.
Advantages
- Speedy analysis: BigQuery excels at processing massive datasets quickly, giving you insights in a flash.
- Real-time analytics: Excellent support for real-time data analysis with real-time streaming capabilities.
- Seamless integration: Being a Google product, BigQuery integrates well with other Google services like Drive and Looker.
- ML integration: Native machine learning capabilities with BigQuery ML, offering a more integrated experience than Snowflake’s external ML tool integration.
Disadvantages
- Advanced features: Lacks some advanced warehousing features available in Snowflake, such as Time Travel and Fail-safe.
- Complex query optimization: Requires more effort to optimize complex queries compared to Snowflake’s automated optimization.
- Cost can creep up: BigQuery's pay-as-you-go model can lead to high bills for complex queries or large data volumes.
- Dependency on Google ecosystem: Heavily tied to the Google Cloud ecosystem, limiting flexibility in multi-cloud environments unlike Snowflake.
Should you consider GBQ?
For budget-focused organizations heavily invested in the Google Cloud Platform (GCP) with bursty query patterns, GBQ's pay-as-you-go model and potential speed advantages could translate to significant cost savings. However, Snowflake remains the ideal choice for cloud neutrality and advanced features like micro-partitioning for complex data structures. Existing Snowflake users might also find GBQ's interface less familiar.
Microsoft Azure Synapse Analytics
.png)
Azure Synapse Analytics is an integrated analytics service that accelerates time to insight across data warehouses and big data systems.
For businesses on Azure, Microsoft Azure Synapse Analytics is a strong alternative to Snowflake. Synapse offers a unified analytics platform (data warehousing, processing, and analytics) with flexible pricing (dedicated or serverless options) and deep Azure integration, potentially reducing costs and streamlining data workflows within the Microsoft cloud.
Key features
- Unified analytics: Combines big data and data warehousing, similar to Snowflake’s unified platform for structured and semi-structured data.
- Synapse Studio: Integrated workspace for data preparation, management, and serving, comparable to Snowflake’s web interface for data management.
- On-demand querying: Combines on-demand and provisioned resources, unlike Snowflake’s single, scalable architecture of virtual warehouses.
- Integrated security: Comprehensive security features, like Snowflake’s robust security measures.
- Data integration: Native integration with Azure Data Factory, Data Lake Storage, and Power BI, akin to Snowflake’s seamless integration with various data and BI tools.
Advantages
- Unified platform: Combines data warehousing and big data analytics in one platform, similar to Snowflake’s support for diverse data types.
- Flexibility: Offers both on-demand and provisioned resources, providing more options for workload management compared to Snowflake’s virtual warehouses.
- Integrated tools: Extensive integration with other Azure services and tools, offering a broader ecosystem for Microsoft-centric environments.
Disadvantages
- Complexity: Can be complex to set up and manage compared to Snowflake’s straightforward setup.
- Performance variability: Performance may vary depending on configuration and workload, whereas Snowflake provides consistent performance.
- Less mature data sharing: Data sharing features are less mature compared to Snowflake’s advanced secure data sharing capabilities.
- Learning curve: Steeper learning curve due to the combination of multiple services and tools, unlike Snowflake’s unified platform.
Should you consider Azure Synapse?
Azure Synapse integrates well with the Microsoft ecosystem and may be cost-effective for those with Azure commitments. However, Snowflake stands out for its superior data sharing capabilities, allowing secure sharing of live data across accounts without moving it. Snowflake also offers more flexible scaling, a simpler usage-based pricing model, and true multi-cloud support, enabling workload mobility across different cloud providers.
Does Snowflake compete with Azure?
Yes, Snowflake competes with Azure Synapse Analytics, particularly in cloud-native analytics. While Snowflake offers multi-cloud support and excels in advanced features like data sharing and elasticity, Synapse leverages its integration within the Azure ecosystem to provide a unified solution for analytics, warehousing, and big data processing.
Data lake + warehousing platforms
Microsoft Azure Data Lake Storage
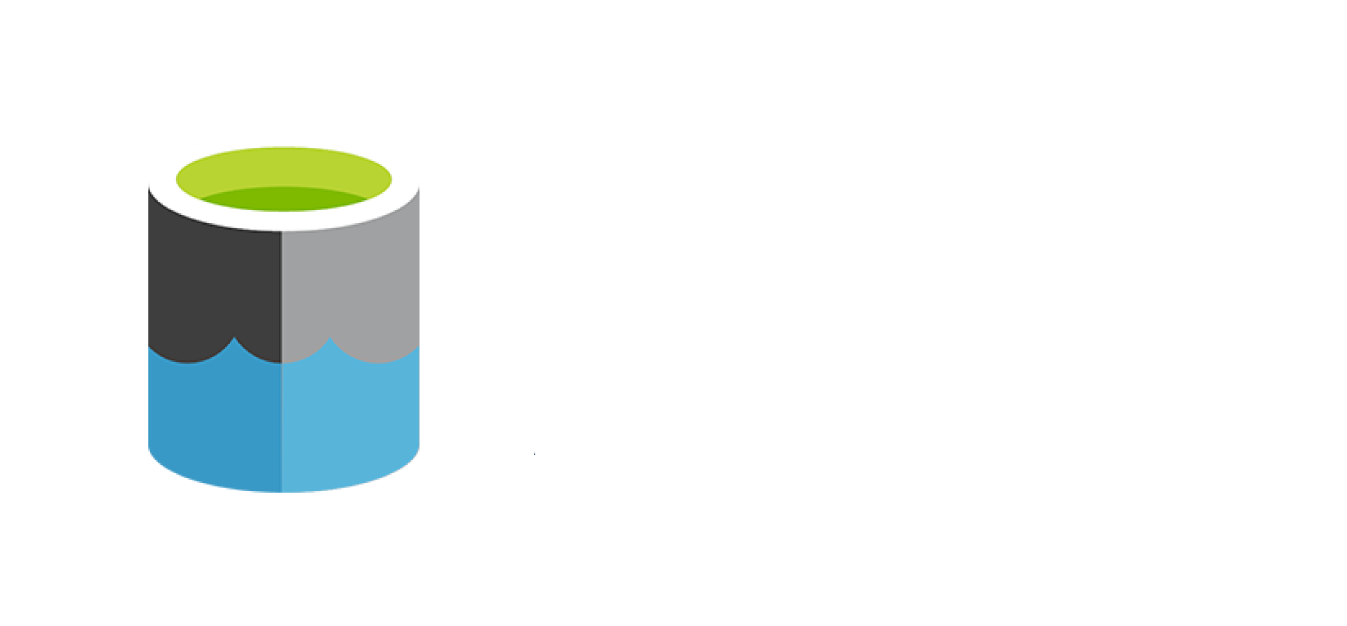
Azure Data Lake Storage is a scalable and secure data lake for high-performance analytics.
Snowflake focuses on structured data analysis, so if you need raw data storage for all types (structured, semi-structured, unstructured) at a lower cost, Microsoft Azure Data Lake Storage (ADLS) is a strong alternative. ADLS acts as a central hub for raw data before processing and integrates well with Azure data tools for a streamlined workflow within the Microsoft cloud.
Key features
- Scalability: Handles massive amounts of data, similar to Snowflake’s ability to scale storage and compute independently.
- Security: Comprehensive security features with encryption and access control, akin to Snowflake’s robust security measures.
- Integration: Integrates with Azure analytics services and tools, comparable to Snowflake’s integrations with various analytics platforms.
- Hierarchical namespace: Organizes data with a hierarchical file system, unlike Snowflake’s flat storage architecture.
- Cost management: Tiered storage options to optimize costs, whereas Snowflake offers cost management through separate storage and compute billing.
Advantages
- Cost-effective storage: Optimized for storing large volumes of data at low cost, providing a more cost-effective solution for data lakes than Snowflake.
- Azure ecosystem: Seamless integration with Azure analytics and AI services, offering a comprehensive data solution within the Microsoft ecosystem.
- Scalability: Scales to accommodate vast amounts of data, ensuring high performance for large datasets.
Disadvantages
- Data management: Requires robust data management and governance practices, whereas Snowflake offers built-in data governance tools.
- Complex querying: Querying capabilities may not be as advanced as Snowflake’s high-performance SQL engine.
- Manual optimization: Requires manual optimization for performance, unlike Snowflake’s automated optimization features.
- Limited cross-cloud support: Primarily designed for Azure, with limited multi-cloud capabilities compared to Snowflake.
Should you consider ADLS?
Azure Data Lake Storage (ADLS) is a cost-effective, scalable choice for large volumes of structured and unstructured data, especially if you're invested in the Azure ecosystem. It offers strong integration with Azure services and fine-grained access control. Opt for Snowflake if you need a comprehensive, cloud-agnostic data warehousing solution with powerful querying capabilities, automatic scaling, and unique data sharing features. Snowflake excels in separating storage and compute resources, allowing flexible scaling, and offers superior data sharing capabilities across different accounts.
Databricks Lakehouse

Databricks Lakehouse combines the best features of data lakes and data warehouses.
If you work with mixed data formats and need a more streamlined workflow, Databricks Lakehouse could be a good alternative to Snowflake. Unlike Snowflake, it stores all your data, organized or not, in one place. This flexibility is ideal for businesses working with diverse data sources.
Key features
- Unified data platform: Combines data engineering, data science, and data analytics, similar to Snowflake’s unified data platform.
- Delta lake: Provides reliability and performance improvements on data lakes, akin to Snowflake’s micro-partitioning for performance optimization.
- Collaborative workspace: Integrated environment for data teams, comparable to Snowflake’s secure data sharing and collaboration features.
- ML and AI integration: Built-in support for machine learning and AI, whereas Snowflake integrates with external ML tools.
- Auto-scaling: Automatically scales compute resources based on workload, similar to Snowflake’s scalable virtual warehouses.
Advantages
- Collaborative environment: Excellent for teams working on data engineering and machine learning, providing a more integrated experience than Snowflake’s external tool integrations.
- Delta lake integration: Enhances reliability and performance of data lakes, offering robust data management features.
- Unified platform: Bridges the gap between data lakes and warehouses, providing a versatile solution for diverse data needs.
Disadvantages
- Learning curve: Steeper learning curve compared to Snowflake’s user-friendly interface.
- Management overhead: Requires more management and optimization, whereas Snowflake offers near-zero maintenance.
- Cost variability: Costs can vary significantly based on usage, similar to Snowflake’s usage-based pricing model.
- Complex setup: More complex to set up compared to Snowflake.
Should you consider Databricks Lakehouse?
Choose Databricks Lakehouse for a unified platform handling data engineering, machine learning, and analytics, especially for large-scale data processing and AI/ML projects. It excels with both structured and unstructured data and integrates well with Apache Spark for big data processing. Opt for Snowflake if you prioritize ease of use, immediate querying capabilities, and seamless data sharing. Snowflake's separated storage and compute architecture allows flexible scaling and cost-effective operations for certain workloads.
Dremio
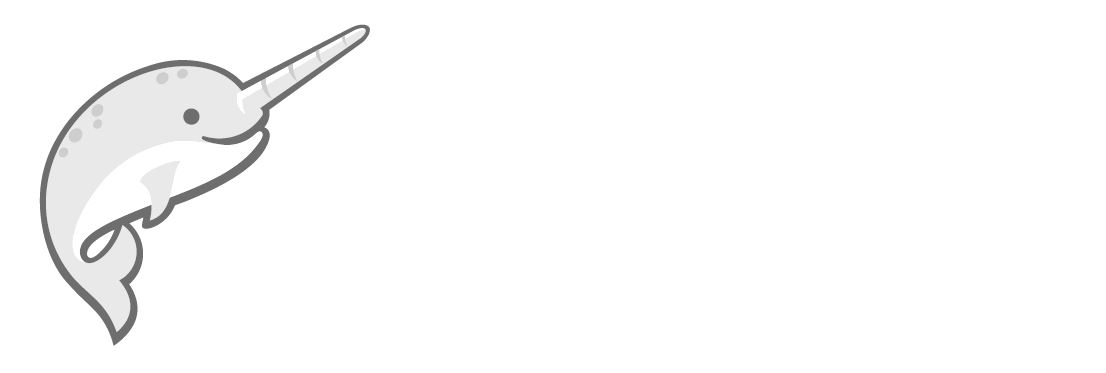
Dremio is a modern data lakehouse platform designed to simplify data analytics by enabling fast and efficient data access across various sources.
Dremio shines as a good alternative to Snowflake for users seeking faster data access and collaboration. While both handle warehousing large datasets, Dremio focuses on accelerating data exploration through features like data reflections (pre-processed data) and a user-friendly interface. This can be more cost-effective for workloads where fast querying of existing data is key, especially for teams working together on analysis.
Key features
- Unified analytics engine: High-performance SQL queries on data lake storage.
- Columnar cloud cache (C3): Accelerates queries by caching data.
- Pushdown optimization: Enhances performance by reducing data movement.
- Data reflections: Speeds up queries with pre-computed views.
- Fine-grained access control: Role-based permissions.
- Broad data source support: Connects to cloud storage, databases, and warehouses.
- Apache arrow and Parquet support: Efficient data storage and high performance.
Advantages
- Self-service analytics: Dremio cuts the IT dependency cord. Your team can query and analyze data directly from various sources data lakes (S3, ADLS) and even Snowflake itself. This translates to faster insights and less time spent waiting on IT.
- Data lake integration: Stuck with a data lake overflowing with potential? Dremio lets you tap into that data directly, ditching the need for complex pipelines to move it all to Snowflake. This can save you time and resources, especially for simpler use cases.
- Data lakehouse architecture: Dremio embraces the data lakehouse approach, bridging the gap between lakes and warehouses. It offers schema management, data governance, and querying capabilities, making your data lake a reliable source for analysis.
- Cost optimization: Dremio can potentially help you optimize costs by minimizing the amount of data stored directly in Snowflake. You can store critical data in Snowflake for complex tasks, while querying the rest from its original source through Dremio.
Disadvantages
- Lacks robust warehousing features: Dremio doesn't compete with Snowflake's advanced data warehousing capabilities. Snowflake is a powerhouse for handling massive, complex datasets and heavy-duty workloads.
- Focus on data access and exploration: Dremio prioritizes user-friendly access and querying across diverse data sources. If you need advanced data warehousing functions like complex transformations or high-performance analytics, Snowflake reigns supreme.
Should you consider Dremio?
If you crave self-service analytics, data lake integration, or cost optimization for specific use cases, Dremio can be a powerful addition to your Snowflake environment. If you're new to data warehousing and prioritize user-friendliness, data lake integration, and cost-effectiveness for simpler needs, Dremio can be a great starting point. However, if you anticipate massive datasets and complex workloads down the line, consider Snowflake's scalability.
Data readiness platform
5X
.png)
The most crucial metric for data teams isn't the volume of data collected, query execution speed, or pipeline uptime. It's actually data readiness.
Data readiness ensures your clean, structured, and centrally modeled data is readily available for a number of use cases: traditional BI, advanced analytics, data activation, and increasingly, AI. It's the foundation for AI and Large Language Models (LLMs) – after all, your AI is only as good as the data it learns from.
Snowflake is great at managing and storing tons of data and is adding cool new features. But is it the full package for getting your data ready? Let’s check it out!
The five layers of a data-ready system are:
- Ingestion
- Warehouse
- Modeling
- Orchestration
- Business Intelligence
Other Considerations
- Total cost of ownership (TCO): Building data pipelines in Snowflake requires multiple services, increasing costs. 5X's integrated platform reduces TCO by 30-50%, simplifying billing and management.
- Integrated services offering: Snowflake often needs extra resources for pipeline management, such as consultants or in-house teams. 5X’s services cost about 25% of US consultancies and 70% of an in-house team.
Is there a free version of Snowflake?
Yes, Snowflake offers a free trial with credits that allow users to explore its platform without upfront costs. This is ideal for businesses testing its capabilities before committing to a paid plan. However, for sustained use, Snowflake does not provide a permanently free version.
Verdict
Snowflake excels as a data warehouse but lacks in comprehensive data readiness, including ingestion, modeling, orchestration, and business intelligence.
5X offers a complete data readiness platform, ensuring your data is clean, accessible, and actionable. Using 5X with Snowflake creates a powerful data stack.
Building a data platform doesn’t have to be hectic. Spending over four months and 20% dev time just to set up your data platform is ridiculous. Make 5X your data partner with faster setups, lower upfront costs, and 0% dev time. Let your data engineering team focus on actioning insights, not building infrastructure ;)
Book a free consultationHere are some next steps you can take:
- Want to see it in action? Request a free demo.
- Want more guidance on using Preset via 5X? Explore our Help Docs.
- Ready to consolidate your data pipeline? Chat with us now.
Wait!
Don't you want to learn how to quickly spot high-yield opportunities?
Discover MoonPay’s method to identify and prioritize the best ideas. Get their framework in our free webinar.
Save your spot

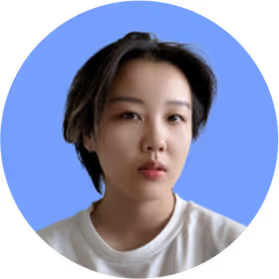