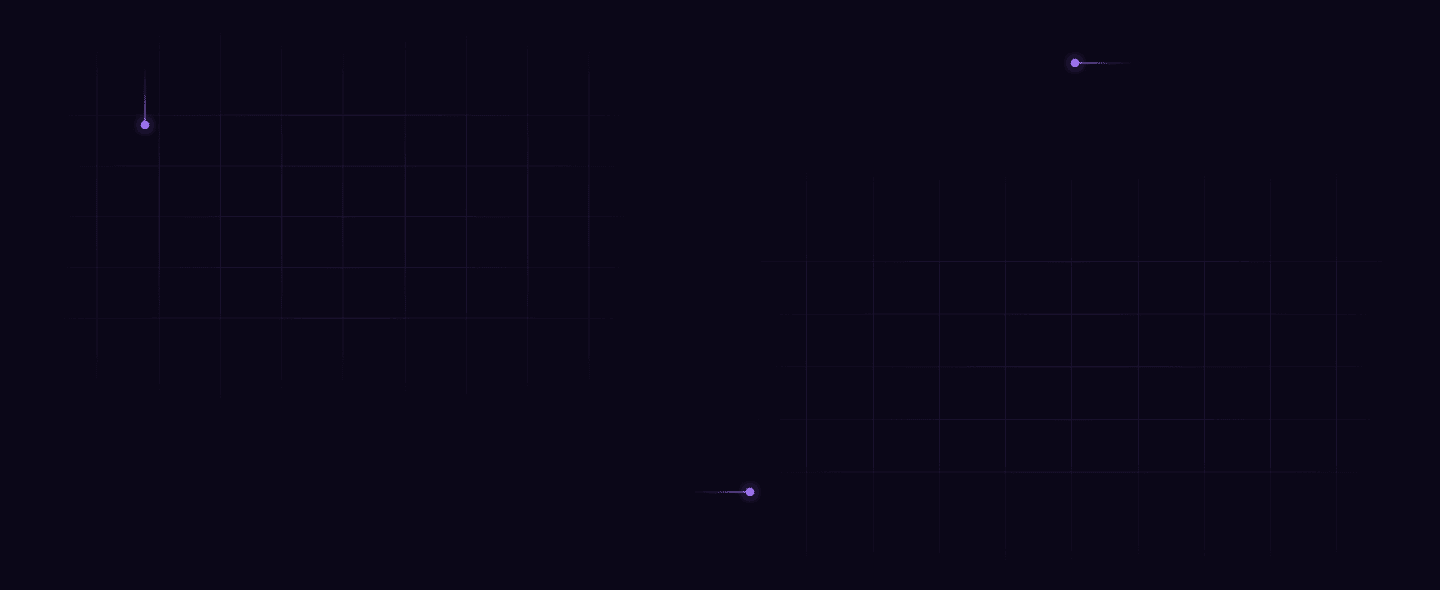
The Business Case for High-Quality Data: A Breakdown of How Data Quality Impacts Your Business
%20(1)%20(1)%20(2).png)
Poor data quality is a massive financial burden, costing businesses an estimated $3 trillion annually in the US alone. Flawed, incomplete, or outdated data also results in misallocated resources, lost opportunities, and operational inefficiencies.
The numbers speak for themselves. Yet, many organizations underestimate the damage that poor data quality can do until it’s too late. In a world where every decision is data-driven, ensuring accuracy and consistency is not optional; it’s crucial for business success.
Read this blog to learn the impact of poor data quality, what causes it, and the steps you can take to prevent data quality issues before they hurt your bottom line.
Good data quality vs. poor quality data: What’s the difference?
Good data quality is data that’s clean, reliable, accurate, up-to-date, and insightful. Good quality data is built from reliable sources and the data is complete with little to zero errors in data.
When data is clean, it builds trust. It makes decision-making seamless. And most importantly, it turns insights into action. That’s why good quality data is the foundation of every solid business decision.
Here are some attributes of good data quality:
- Accurate
- Consistent
- Up-to-date
- Relevant to your business
- Available to key stakeholders
- Accessible
- Secure
Poor data quality is data that’s inaccurate, incomplete, inconsistent, or outdated. It fails to meet the standards required for effective business operations. It sends companies chasing after phantom opportunities or scrambling to fix avoidable mistakes.
Here are some attributes of poor data quality:
- Inaccurate
- Inconsistent
- Incomplete
- Sourced from unreliable sources
- Not vetted or verified
What causes poor data quality?
Poor data quality can result from human errors, poor data governance, lack of validation processes, system mismatches, or fragmented data sources.
- Inconsistent data collection methods
- Ineffective data management
- Outdated or stale data
- Not verifying and fixing data errors at the source
1. Inconsistent data collection methods
When data is entered without uniform standards, errors creep in. If your CRM, invoicing system, or analytics platform allows inconsistent inputs, the result is unreliable information.
Make sure that you standardize your data entry processes across all touchpoints.
With clear guidelines in place, businesses ensure that data remains accurate, structured, and usable—saving time, effort, and costly corrections down the road
2. Ineffective data management
Lack of governance around data handling can create confusion.
When different departments define, format, and manage data independently without consulting each other, silos emerge, making it difficult to share insights or maintain accuracy. This issue worsens when organizations undertake complex tasks like data migration or integration.
Establishing a clear framework for data management ensures consistency and reliability across the board. After standardizing records, companies must also invest in training employees across departments to effectively read, interpret, and leverage data.
3. Outdated or stale data
Even the best data loses its value if it’s not updated.
Businesses that collect information but fail to update it regularly risk making decisions based on outdated records. Expired email addresses, incorrect customer details, or obsolete transaction histories can lead to missed opportunities and flawed strategic planning. Keeping data current ensures that it remains a reliable asset rather than a liability.
By tackling these challenges head-on, organizations can shift from reactive data cleanups to proactive data excellence. This fuels better decisions, seamless operations, and sustainable growth.
4. Not verifying and fixing data errors at source
Bad data doesn’t just appear — it’s the result of teams overlooking missing information or failing to verify unusual data points. Instead of investigating discrepancies, employees under pressure often make quick fixes, treating errors as minor roadblocks rather than systemic issues.
These mistakes don’t just stay in one place; they spread.
For example, when Team B quietly corrects errors instead of flagging them to Team A, the cycle continues. Without feedback, Team A remains unaware of the flaws in their data, missing the opportunity to diagnose and resolve the underlying issues.
These unchecked errors multiply creating inefficiencies over time.
The true cost of poor data quality: How it impacts your business
Poor data quality slows things down and derails entire operations.
It’s a silent disruptor lurking beneath every spreadsheet, CRM system, and business process. And yet, many companies underestimate the damage it can do, often paying for it in precious dollars.
Here’s a quick overview of how poor data quality affects your business outcomes:
- Faulty decision making
- Inefficient operations
- Poor customer experience
- Compliance risks and penalties
- Revenue loss and missed opportunities
1. Faulty decision making
According to a Forbes Insights and KPMG report, 84% of CEOs have expressed concern about the quality of data they use to make decisions. This highlights the widespread worry among top executives regarding the reliability of their data-driven strategies.
This fear is not unfounded. After all, relying on poor data quality can result in strategic errors such as targeting the wrong audience, investing in off-base markets, or allocating resources inefficiently.
2. Inefficient operations
Inaccurate data leads to wasted time and resources. Employees may spend hours correcting errors, manually reconciling discrepancies, or dealing with system failures.
Here are some examples:
- Sales teams lose valuable hours chasing leads with incorrect contact details or outdated prospect information
- Service delivery people waste time correcting flawed customer orders received from sales
- Data scientists waste precious time cleaning and organizing messy data rather than generating insights that drive innovation
- IT teams work overtime to align disconnected systems that weren’t built to communicate, leading to inefficiencies and increased costs
- Senior executives hesitate to make bold strategic decisions because they don’t fully trust the numbers coming from finance
3. Poor customer experience
Bad data can cause incorrect billing, miscommunication, or irrelevant recommendations, frustrating customers and reducing retention rates.
This is particularly relevant for an eCommerce company where every decision you make, starting with the products you sell to your app’s UI/UX, to the data you make available, matters.
4. Compliance risks and penalties
In industries with strict regulations such as in finance and healthcare, inaccurate data can lead to non-compliance, resulting in hefty fines or legal action.
For example, in banking and financial services, KYC (Know Your Customer) and AML (Anti-Money Laundering) regulations require accurate customer identification and transaction monitoring.
If a bank maintains incomplete or inconsistent customer records, it may fail to detect fraudulent transactions, inadvertently facilitating financial crimes. This can result in multi-million-dollar penalties such as in 2020, when Deutsche Bank was fined $150 million for failing to maintain accurate records and detect suspicious transactions linked to sex offender Jeffrey Epstein.
5. Revenue loss and missed opportunities
Gartner measures the average financial impact of poor data on businesses at $9.7 million per year. These costs are not solely financial as businesses also face missed opportunities.
Beyond financial losses, a company may miss a critical opportunity for new product development or customer need that a competitor with a more mature understanding of data may capitalize upon.
6. Reputational damage
In some cases, your reputation may be on the line when you operate in a public-facing industry.
For example, poor data in banking could lead to inadvertent trade with sanctioned governments or suspected terrorist financiers if institutions don't have accurate enough information about the customers they're trading with, resulting in PR fallout on top of punitive fines.
How to prevent and troubleshoot data quality issues?
The key to preventing poor data quality is recognizing its root causes before they spiral into bigger issues and taking the right precautions in time. Here’s an overview:
- Identify the root cause: Examine whether poor data quality issues arise from human errors, system failures, integration mismatches, outdated records, or discrepancies in third-party data sources. Use system logs and data lineage tracking to identify the root cause.
- Standardize data input processes: Set standardized rules, implement naming conventions, and define required fields and acceptable values. Determine specific formats for key fields (e.g., Use YYYY-MM-DD for dates, standardize address fields, etc.). Additionally, you can implement mandatory validation checks at the point of entry to prevent missing, duplicate, or incorrect data from entering the system.
- Use data cleaning tools: Leverage advanced data quality management tools to remove duplicates, correct inconsistencies, and enrich incomplete records.
- Automate data validation: Use automated tools to detect anomalies, missing values, and duplicates. These tools can flag missing information and inconsistencies, validate records against predefined rules in real-time, and prevent poor data from entering your system.
- Regular data audits: Schedule routine data audits to identify inconsistencies and potential risks. Conducting periodic reviews helps detect problems before they escalate.
- Implement a business risk matrix: Assess how data quality issues impact different areas of your business. A business risk matrix can help prioritize fixes based on its severity and potential impact. For instance, incorrect customer contact details may lead to minor inconvenience, while financial data errors could result in significant regulatory penalties. Categorizing issues by severity ensures that critical problems are addressed immediately while lower-priority discrepancies are managed systematically.
- Improve data monitoring and reporting: Set up real-time monitoring dashboards to track data health, flag discrepancies, and take immediate action before issues escalate.
- Strengthen data integration across systems: Poor integration between CRM, ERP, and other data systems often leads to misaligned records. Ensure seamless connectivity and synchronization across platforms. Use data integration tools to simplify the process.
- Establish data governance policies: A strong data governance framework ensures data accuracy, consistency, and security. Implement clear guidelines on data entry, validation, and storage across all departments.
- Enhance employee training: Many data errors are made by humans. Provide regular training to employees handling data to ensure they understand best practices and quality standards.
Final thoughts: High-quality data is good for business
Companies that prioritize high-quality data gain a competitive edge, reduce costly errors, and improve customer trust. Those that ignore it risk inefficiency, financial losses, and reputational damage. After all, data quality is the difference between companies that struggle and those that thrive. Between businesses that react and those that lead.
Investing in data quality management, implementing a rigorous data quality process, and fostering a data-driven culture can prime your organization for success.
Don’t let bad data hold your business back. Take control and turn accurate data into your greatest asset. Schedule a demo with our team to learn how we can transform your data strategy today.
What strategies can prevent data quality issues and enhance customer satisfaction?
What are the key challenges in maintaining data integrity, and how can they be resolved?
How can a standardization guide improve data quality and operational efficiency?
Why are analytical skills essential for maintaining high-quality data?
Building a data platform doesn’t have to be hectic. Spending over four months and 20% dev time just to set up your data platform is ridiculous. Make 5X your data partner with faster setups, lower upfront costs, and 0% dev time. Let your data engineering team focus on actioning insights, not building infrastructure ;)
Book a free consultationHere are some next steps you can take:
- Want to see it in action? Request a free demo.
- Want more guidance on using Preset via 5X? Explore our Help Docs.
- Ready to consolidate your data pipeline? Chat with us now.
Table of Contents
Get notified when a new article is released
Get an end-to-end use case built in 48 hours
Get an end-to-end use case built in 48 hours
How retail leaders unlock hidden profits and 10% margins
Retailers are sitting on untapped profit opportunities—through pricing, inventory, and procurement. Find out how to uncover these hidden gains in our free webinar.
Save your spot
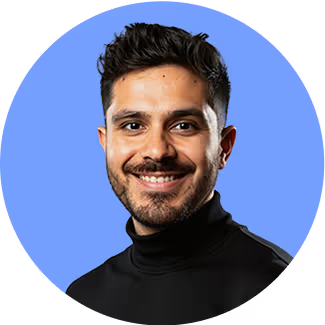